ATP2C2 as a novel immune-related marker that defines the tumor microenvironment in triple-negative breast cancer
Introduction
Breast cancer has recently become the most often diagnosed malignancy in females (1). According to pathological criteria, breast cancer can be subdivided into several subtypes. Triple-negative breast cancer (TNBC) is an aggressive breast subtype that accounts for about 10–20% of all mammary cancers. It lacks estrogen receptor (ER), progesterone receptor (PR), and human epidermal growth factor receptor 2 (HER2) (2). Epidemiological studies reveal that TNBC occurs predominantly in premenopausal women (3). TNBC is characterized by a high malignancy, strong invasiveness, early metastasis, and short survival time (4). Patients with TNBC are often resistant to endocrine and molecular targeted therapy. In addition, the pathogenesis of TNBC is still poorly understood, making clinical treatment challenging. At the moment, surgery and systemic chemotherapy remain the primary line of defense against TNBC (4,5).
The tumor microenvironment has a crucial role in infinite proliferation, tumor invasion, angiogenesis, and even immune evasion. Recent researches have shown that tumor microenvironment (TME)-related immune scores and the estimation of the proportion of tumor-infiltrating immune cells (TICs) in the TME are useful for assessing the prognosis of treatments and may be promising in optimizing future targeted therapies (6-8). For example, TME-related immune scores have been demonstrated to be effective predictors of recurrence in colorectal cancer, and an international consensus has been reached about the efficacy of using ImmuneScore to classify colorectal cancer (9,10). Therefore, identifying new TME-associated biomarkers may be very useful for differentiating breast cancer subtypes.
Immunotherapy drugs, such as programmed cell death protein 1 (PD-1) or programmed cell death-ligand 1 (PD-L1) immune checkpoint inhibitors, have been studied in over a thousand of clinical trials and approved for the treatment of a variety of cancers (11,12). The aim of this study was to discover TME-related biomarkers that can predict prognosis and immunotherapy efficacy using bioinformatics analysis. Genes related to TME were investigated using the expression profile and clinical data of TNBC patients from the Gene Expression Omnibus (GEO) database with several bioinformatics tools. Analyses revealed that ATPase Secretory Pathway Ca2+ Transporting 2 (ATP2C2) may be associated with the development of TNBC. There is evidence that ATP2C2, Kv10.1, and Orai1 are implicated in TME cell signal transduction, indicating that ATP2C2 may have a significant role in TME (13). Thus, ATP2C2 may potentially serve as a new biomarker for TNBC. This article is presented in accordance with the TRIPOD reporting checklist (available at https://tcr.amegroups.com/article/view/10.21037/tcr-23-83/rc).
Methods
TNBC datasets and resources
mRNA expression data and relevant clinical information from samples with TNBC (breast cancer negative for ER, PR, and HER2) were downloaded from GEO datasets (GSE58812 and GSE25055). 107 TNBC samples from the GSE58812 were used as the training cohort to generate the prognostic signature, while the 113 TNBC samples from the GSE25055 were utilized to validate the prognostic signature of the validation cohort.
Patients and samples
Twenty paired paraffin specimens that including tumor and adjacent tissues from TNBC patients at the Zhejiang Hospital were gathered. All patients were diagnosed as breast cancer and underwent surgical resection. The ER (−), PR (−), and HER2 (−) status of the TNBC patients were determined according to the results of immunohistochemistry (IHC) by the Pathology Department of Zhejiang Hospital. This study was conducted in compliance with the Declaration of Helsinki (as revised in 2013) and was authorized by the Medical Ethics Committee of Zhejiang Hospital [No. 2022(79K)-X1]. The participants gave informed consent before taking part in the study.
Associations of ImmuneScore, StromalScore, and ESTIMATEScore with survival rate
The stromal, immune, and ESTIMATE scores were outputted by the R package “estimate”. The patients were separated into two groups according to the quartiles of ImmuneScore, StromalScore, and the median of ESTIMATEScore. Then, the Kaplan-Meier (K-M) survival curve was constructed using “Survival” and “SurvMiner” software packages between the two group of patients by log-rank test. All P values were two-sided, and the results were considered statistically significant when the P values were <0.05.
TME-related DEGs identified
DEGs were identified in the low and high ImmuneScore groups and in the low and high ESTIMATEScore groups using the “Limma” R package. The criteria for DEGs were P<0.05 and |logFC| >0.5.
Identification and construction of prognosis-related signature models
Univariate Cox regression was performed on potential prognostic TME-related genes associated with the survival time of TNBC patients and three prognostic genes were established with P<0.05. Subsequently, multivariate Cox regression was performed to construct TME-related gene signature and calculated TMErisk score. The TMErisk score was established based on a linear combination of expression levels of TME-related genes weighted by the regression coefficients that were calculated by log transformation of the hazard ratio (HR) from the multivariate Cox regression. TMErisk score was established with the following formula: Risk score = (exprgene1 × Coefgene1) + (exprgene2 × Coefgene2) + … + (exprgenen × Coefgenen) (14). TNBC patients were then divided into high- and low-risk groups on the basis of the median of the TMErisk score. K-M survival curves and time-dependent ROC curves were drawn and used to evaluate the efficiency of the TMErisk model.
ATP2C2 differential expression and survival analyses
The website (http://gepia.cancer-pku.cn/) provides information on the expression of ATP2C2 in various malignancies. BRCA seq-counts were downloaded from UCSC Xena (https://xenabrowser.net/datapages/). Sample counts were compared to determine the differences between TNBC and normal samples. Histogram results were drawn on Xiantao Academic website. Survival data for high and low ATP2C2 expression in BRCA are available on the GEPIA website (http://gepia.cancer-pku.cn/).
Z-score was utilized to process the ATP2C2 expression quantity of the training cohort. The processed data ≥1 were separated into the high expression group, whilst those <1 were separated into the low expression group. The verification cohort was separated into groups of high and low expression of ATP2C2 based on the mean of ATP2C2 expression. K-M survival curve was plotted using “Survival” and “SurvMiner” R software packages. Log-rank test was used to compare the groups of high and low expression of ATP2C2, and P<0.05 was considered significant.
Evaluation of TICs
The proportions of TICs in each TNBC patient were calculated using “xCell” R package. Wilcoxon rank-sum test and Pearson’s correlation analysis were used to evaluate the fraction of tumor immune cells with low and high ATP2C2 expression. Results were shown in box plot and scatter plots using “ggplot2” and “ggpubr” R packages.
Gene set enrichment analysis (GSEA)
GSEA analysis was implemented using the R package “clusterProfiler” between low- and high-ATP2C2 expression (15). Significant enrichment pathway was identified using normalized enrichment score (|NES| >1, FDR q-value <0.01, and P<0.01.
Immunohistochemistry
Samples from 20 TNBC patients admitted to our institution were used for immunohistochemical examination (samples were embedded in paraffin and sectioned). Briefly, 3-µm-thick sections were cut, transferred on glass slides (Zhongshan, ZLI-9506, Beijing, China), toasted at 70 ℃, and dewaxed. Sections were then incubated in Tris/EGTA buffer (pH=9) and treated with Rabbit polyclonal ATP2C2 antibody (1:200, NBP2-14329, NOVUS) at 4 ℃ overnight, followed by incubation with peroxidase-conjugated anti-rabbit IgG (1:1,000, DAKO, Denmark) at 37 ℃ for 30 min. Lastly, the reaction was conducted for 2 min using a BAD staining solution (DM827, DAKO, Denmark).
Statistical analysis
Rstudio (version 4.0.0) was used for the majority of statistical studies, including differential expression gene (DEGs) analysis, principal component analysis (PCA), univariate and multivariate Cox regression models, K-M survival analysis, and ROC curve analysis. The quantitative information was reported as mean ± standard deviation (SD). Wilcoxon test was used to compare the two groups, and P<0.05 was considered to be statistically significant.
Results
ImmuneScore and ESTIMATEScore were associated with the survival of TNBC
Initially, the association between immune infiltration score and prognosis of TNBC patients was evaluated. Patients were classified into low and high groups according to the quartile of ImmuneScore and StromalScore, and the median of ESTIMATEScore, respectively. Survival status and immune infiltration scores of samples from 107 patients were combined to generate a K-M survival curve. The results revealed that patients with a high ImmuneScore and ESTIMATEScore had a higher survival time than those with a low score (P=0.044 and P=0.017, respectively; Figure 1A,1B). However, StromalScore was not associated with survival time (Figure 1C). These findings imply that the immunological component of the TME is a potentially favorable prognostic factor for TNBC patients.
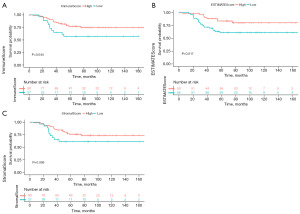
DEGs of high and low ImmuneScore, ESTIMATEScore groups
To establish the association between gene expression and ImmuneScore, ESTIMATEScore, gene expression differences were analyzed between groups with high and low ImmuneScore and ESTIMATEScore. There were 346 DEGs between the low vs. high ImmuneScore groups and 241 DEGs between the low vs. high ESTIMATEScore groups (Figure 2A,2B). In addition, 57 DEGs were shared by ImmuneScore and ESTIMATEScore, including 26 up-regulated genes and 31 down-regulated genes (Figure 2C). These genes may have been the primary determinants of the TME’s status in TNBC.
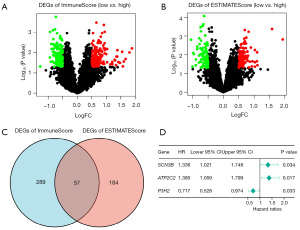
Screening of TME-related genes and construction of TMErisk score
Transcriptional information and clinical data of TNBC patients were integrated to investigate the association of TME-related genes with prognosis in TNBC patients. Firstly, the 57 DEGs were used to identify TME-related prognostic genes using univariate COX regression analysis, and the condition of prognosis-related genes was P<0.05. Three related prognostic genes were found, including 1 gene (P3H2) associated with favorable survival and 2 genes (SCN3B, ATP2C2) with unfavorable survival (Figure 2D). A multivariate Cox regression analysis identified three genes as strongly TME-related genes. Based on expression scores and risk coefficients of the three TME-related genes (Table S1), TMErisk score was calculated. The TMErisk score of each patient was calculated as follows: TMErisk = (0.30637*SCN3B) + (0.27001*ATP2C2) + (−0.33951*P3H2). Patients were divided into high-risk (n=53) and low-risk (n=54) groups based on the median cut-off value of the TMErisk score (Figure 3A). It was worth noting that the number of deaths was significantly lower in the low-risk group (Figure 3B). A heatmap displayed that patients in the high-risk group tended to show increase expression of ATP2C2 and SCN3B, as well as decrease expression levels of P3H2 (Figure 3C). The K-M survival curve and log-rank tests revealed that TNBC patients in the high-risk group had a lower survival rate than those in the low-risk group, with 60-month survival rate (high-risk group and low-risk group) of 60% and 80%, respectively (Figure 3D). The ROC curve further demonstrated that AUC values of 3- and 5-year had a strong predictive capacity, with AUC values of 0.655 and 0.727, respectively (Figure 3E).
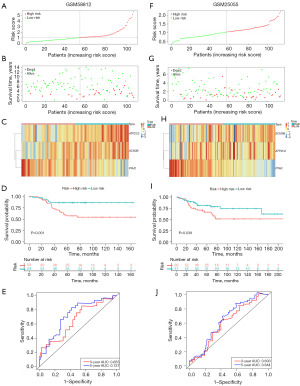
Validation of the TMErisk signature
To validate that the TMErisk model has alike predictive value in different crowds, the GSE25055 dataset was used to validate the survival and ROC curves predicted by the training cohort. The 113 TNBC patients were separated into two groups based on the median value of the TMErisk score: high-risk group (n=56) and low-risk group (n=57) (Figure 3F). The number of death patients (Figure 3G) and gene expression (Figure 3H) were displayed in figures. TNBC samples in the high-risk group had a striking lower survival rate than those in the low-risk group (P=0.039, Figure 3I). The validation cohort also showed the prognostic model with strong prediction ability for 3- and 5-year survival rates (Figure 3J). The above results indicated that the TMErisk signature performed well in forecasting the survival of TNBC patients.
ATP2C2 gene expression and prognosis
GEPIA data revealed variations in the expression of ATP2C2 in several cancers (Figure 4A). In eight malignancies, the expression of ATP2C2 was greater than in the normal group (including breast cancer, Figure 4A). In the TCGA database, the ATP2C2 mRNA level of TNBC patients was higher than that of normal breast tissue (Figure 4B). According to the GEPIA database, breast cancer patients with low ATP2C2 expression had a considerably greater survival rate than those with high expression (Figure 4C).
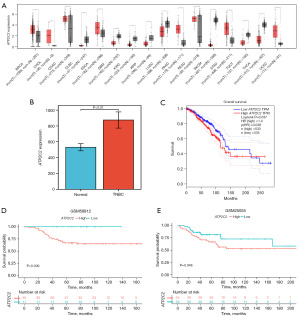
Next, the survival rates of the low and high ATP2C2 groups in the training and validation cohorts were compared and the low ATP2C2 group in TNBC patients was found to have a significantly higher survival rate than the high ATP2C2 group (Figure 4D,4E). ATP2C2 was shown to be a gene that may be utilized to predict the prognosis of TNBC.
The association of ATP2C2 expression and TICs
Using the “xCell” R package, the TICs of TNBC samples were computed to investigate the relationship between ATP2C2 expression and TICs. The results revealed that 16 TICs differed significantly between the high and low ATP2C2 groups (Figure 5A). Eleven kinds of immune cells were higher in the low ATP2C2 group. In addition, three immune scores also were calculated using xCell algorithm, including ImmuneScore, StromalScore, and MicroenvironmentScore. Results showed that only MicroenvironmentScore was statistically significant in relation to the expression of ATP2C2 (Figure 5A). Linear correlation analysis suggested that 10 TICs and MicroenvironmentScore were inversely correlated with ATP2C2 expression (Figure 5B-5L).
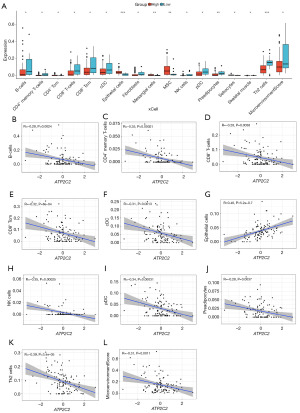
GSEA of ATP2C2
GSEA was implemented to conclude the functional differences between the low and high ATP2C2 expression. Numerous significant pathways were identified to be associated with immunity in the enrichment of MSigDB Collection (c5.all.v7.0.entrez.gmt) in the low ATP2C2 group, such as adaptive immune response, lymphocyte activation, lymphocyte differentiation, positive regulation of immune response, regulation of immune system process, and T cell activation (Figure 6A), while the significant enrichment pathways associated with high ATP2C2 expression were keratinization-related pathways, comprising cornification pathway, keratin filament, keratinization, and skin development (Figure 6B). These findings suggested that ATP2C2 may serve as a biomarker of TME status.
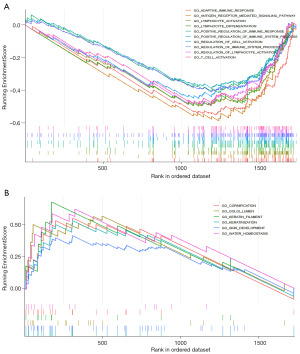
ATP2C2 expression in TNBC and normal tissue
Epithelial cells of the mammary duct-lobular system are double-layered. The innermost layer (luminal layer) is composed of epithelial cells, while the outermost layer (basal layer) is composed of myoepithelial cells. ATP2C2 expressed in the myoepithelial cells and basement membrane (Figure 7A, as shown by the arrow) and weakly expressed in the epithelial cells of the normal mammary gland, as determined by immunohistochemical analysis (Figure 7A). However, ATP2C2 diffusely expressed in tumor cells of TNBC (Figure 7B).
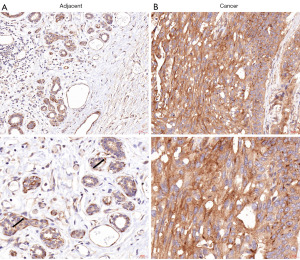
Discussion
The present study aimed to identify genes related to TME that would influence the survival rate of TNBC patients. ATP2C2 has been linked to immunological response and may serve as a biomarker for diagnosing and treating TNBC. The ESTIMATE algorithm was initially employed to estimate tumor purity and identify potential TME-related biomarkers. Then, using the ESTIMATE algorithm to generate ImmuneScore, StromalScore, and ESTIMATEScore in the training cohort, the survival time of TNBC patients was found to be linked with ImmuneScore and ESTIMATEScore. This suggests that TME-related characteristics may predict the prognosis of cancer patients, as documented in a large number of previous studies (16-18).
Second, for differential gene analysis, the ImmuneScore and ESTIMATEScore were divided into low and high groups, and 57 differential genes associated with TME were identified. Next, TME-related genes associated with patients’ prognosis were explored. Univariate Cox regression analysis revealed that P3H2, SCN3B, and ATP2C2 were substantially correlated with survival time. Each patient’s risk score was then calculated using multivariate analysis, and a TMErisk model was constructed. According to the K-M plot analysis, the greater the score of the TMErisk model, the worse the prognosis. Further study revealed that the ATP2C2 gene was the strongest predictor of TNBC survival and prognosis. Therefore, ATP2C2 was selected for further investigation.
ATP2C2 is a protein-coding gene that participates in Ca2+ transport in secretion. ATP2C2 is expressed in breast tissue, gastrointestinal tract, respiratory organs, prostate and salivary glands (19). Several studies found a relationship between ATP2C2 and language impairments (20,21), while other studies indicated an association between ATP2C2 and the prognosis of certain cancers (22-24). One study has demonstrated that ATP2C2 may suppress the migration and metastasis of cancer cells by reversing epithelial-mesenchymal transition (EMT) (25). Nevertheless, it has also been reported that ATP2C2-transfected colon cancer cells exhibit a higher number of cells entering the S phase by regulating the cell cycle (22). Girault et al. demonstrated that the association between Kv10.1, Orai1, and SPCA2 is essential for collagen-induced MCF-7 breast cancer cell survival (26). In these studies, ATP2C2 had contradictory effects on tumor growth and metastasis, which was likely related to the multiple functions that ATP2C2 exerted in tumor growth and development. Studies have also suggested that ATP2C2 is an independent prognostic factor and has a good predictive effect on the survival of thyroid cancer and breast cancer patients (23,24). Patients with high expression of ATP2C2 have a higher mortality rate, which is consistent with our findings.
In recent years, the connection between immune cell infiltration and malignancy has sparked a flurry of research (27,28). This study used the xCell algorithm (29) to assess 64 types of immune cells in the TNBC sample. The score was used to compare the estimated number of immune cells between samples, and its utility has been verified in previous articles (30). According to our findings, the proportion of 11 kinds of immune cells was higher in the low ATP2C2 group than in the high ATP2C2 group, including B cells, T cells (CD4+ memory cells, CD8+ cells, CD8+ Tcm cells, and Th2 cells), conventional dendritic cells (cDCs), fibroblasts, NK cells, plasmacytoid dendritic cells (pDCs), preadipocytes, skeletal muscle. An increased abundance of CD4 and CD8 T cells in tumor-infiltrating lymphocytes (TIL) is associated with better survival outcomes (31,32). Additionally, tumors with a high CD8 and CD4 memory T cell count have been associated with higher survival rates in TNBC patients (33). Steinman, who received the Nobel Prize in Biomedical Sciences in 2011, discovered that dendritic cells (DCs) are the commanding officers of the human immune system, guiding diverse immune system operations (34). DCs are a heterogeneous class of innate immune cells. The dominant DC types contain cDCs, pDCs, and inflammatory DCs (infDCs), which have different ontogeny, immune characteristics, and specific effects. In humans, cDCs can present tumor antigens and secrete cytokines that modulate T cell activation and effector activity to have anti-tumor immune responses. cDCs can be also classified into conventional type 1 dendritic cells (cDC1) and conventional type 2 dendritic cells (cDC2) (35). NK cells are natural killer cells found in the human body that are primarily associated with anti-tumor, anti-viral, immunological control, allergies, and other variables. It has been reported that NK cells recruit cDC1 into the tumor microenvironment, improving cancer immune control. In an independent cohort of breast cancer samples (in the TCGA dataset), Böttcher et al. discovered a comparable positive correlation between NK cell and cDC1 gene signatures. Surprisingly, TNBC samples demonstrated a highly significant positive relationship between signature genes of cDC1 and NK cells and survival (36). These researchers demonstrated the role of NK cells and cDCs in anti-tumor immunity, confirming our findings. The proportion of NK cells and cDCs cells was greater in the low ATP2C2 group, indicating that ATP2C2 might reverse the anti-tumor immunity of NK cells and cDCs. Furthermore, the group with reduced ATP2C2 expression had a greater microenvironment score.
Finally, the ATP2C2 expression of 20 cases of TNBC patients from our hospital from 2018–2022 was investigated in this study. The expression of ATP2C2 was higher in tumors than in adjacent tissues, consistent with the findings in the aforementioned studies. However, because of the limited number of available TNBC samples and the short duration of present of the disease in the included patients, it was impossible to report the condition in a 10-year period; however, we will continue to monitor their survival status of the included patients. Overall, ATP2C2 might become a potential advantageous immunomodulatory agent for TNBC management.
Conclusions
This study showed that ATP2C2 plays an important part associated with immunity activity in the TME of TNBC. ATP2C2 might be a potential biomarker to improve clinic prognosis.
Acknowledgments
We would like to express our gratitude to all researchers and participants for their contributions to this research and thank the public databases, including TCGA, GEO and GEPIA, for providing data.
Funding: This work was supported by
Footnote
Reporting Checklist: The authors have completed the TRIPOD reporting checklist. Available at https://tcr.amegroups.com/article/view/10.21037/tcr-23-83/rc
Data Sharing Statement: Available at https://tcr.amegroups.com/article/view/10.21037/tcr-23-83/dss
Peer Review File: Available at https://tcr.amegroups.com/article/view/10.21037/tcr-23-83/prf
Conflicts of Interest: All authors have completed the ICMJE uniform disclosure form (available at https://tcr.amegroups.com/article/view/10.21037/tcr-23-83/coif). The authors have no conflicts of interest to declare.
Ethical Statement: The authors are accountable for all aspects of the work in ensuring that questions related to the accuracy or integrity of any part of the work are appropriately investigated and resolved. This study was conducted in compliance with the Declaration of Helsinki (as revised in 2013) and was authorized by the Medical Ethics Committee of Zhejiang Hospital [No. 2022(79K)-X1]. The participants gave informed consent before taking part in the study.
Open Access Statement: This is an Open Access article distributed in accordance with the Creative Commons Attribution-NonCommercial-NoDerivs 4.0 International License (CC BY-NC-ND 4.0), which permits the non-commercial replication and distribution of the article with the strict proviso that no changes or edits are made and the original work is properly cited (including links to both the formal publication through the relevant DOI and the license). See: https://creativecommons.org/licenses/by-nc-nd/4.0/.
References
- Sung H, Ferlay J, Siegel RL, et al. Global Cancer Statistics 2020: GLOBOCAN Estimates of Incidence and Mortality Worldwide for 36 Cancers in 185 Countries. CA Cancer J Clin 2021;71:209-49. [Crossref] [PubMed]
- Wolff AC, Hammond ME, Hicks DG, et al. Recommendations for human epidermal growth factor receptor 2 testing in breast cancer: American Society of Clinical Oncology/College of American Pathologists clinical practice guideline update. J Clin Oncol 2013;31:3997-4013. [Crossref] [PubMed]
- Howard FM, Olopade OI. Epidemiology of Triple-Negative Breast Cancer: A Review. Cancer J 2021;27:8-16. [Crossref] [PubMed]
- Yin L, Duan JJ, Bian XW, et al. Triple-negative breast cancer molecular subtyping and treatment progress. Breast Cancer Res 2020;22:61. [Crossref] [PubMed]
- Schmid P, Cortes J, Dent R, et al. Event-free Survival with Pembrolizumab in Early Triple-Negative Breast Cancer. N Engl J Med 2022;386:556-67. [Crossref] [PubMed]
- Yang S, Liu T, Nan H, et al. Comprehensive analysis of prognostic immune-related genes in the tumor microenvironment of cutaneous melanoma. J Cell Physiol 2020;235:1025-35. [Crossref] [PubMed]
- Janes PW, Vail ME, Ernst M, et al. Eph Receptors in the Immunosuppressive Tumor Microenvironment. Cancer Res 2021;81:801-5. [Crossref] [PubMed]
- So JY, Ohm J, Lipkowitz S, et al. Triple negative breast cancer (TNBC): Non-genetic tumor heterogeneity and immune microenvironment: Emerging treatment options. Pharmacol Ther 2022;237:108253. [Crossref] [PubMed]
- Bruni D, Angell HK, Galon J. The immune contexture and Immunoscore in cancer prognosis and therapeutic efficacy. Nat Rev Cancer 2020;20:662-80. [Crossref] [PubMed]
- Yue Y, Zhang Q, Sun Z. CX3CR1 Acts as a Protective Biomarker in the Tumor Microenvironment of Colorectal Cancer. Front Immunol 2022;12:758040. [Crossref] [PubMed]
- Sun C, Mezzadra R, Schumacher TN. Regulation and Function of the PD-L1 Checkpoint. Immunity 2018;48:434-52. [Crossref] [PubMed]
- Bianchini G, De Angelis C, Licata L, et al. Treatment landscape of triple-negative breast cancer - expanded options, evolving needs. Nat Rev Clin Oncol 2022;19:91-113. [Crossref] [PubMed]
- Peretti M, Badaoui M, Girault A, et al. Original association of ion transporters mediates the ECM-induced breast cancer cell survival: Kv10.1-Orai1-SPCA2 partnership. Sci Rep 2019;9:1175. [Crossref] [PubMed]
- Luan F, Chen W, Chen M, et al. An autophagy-related long non-coding RNA signature for glioma. FEBS Open Bio 2019;9:653-67. [Crossref] [PubMed]
- Yu G, Wang LG, Han Y, et al. clusterProfiler: an R package for comparing biological themes among gene clusters. OMICS 2012;16:284-7. [Crossref] [PubMed]
- Pagès F, Mlecnik B, Marliot F, et al. International validation of the consensus Immunoscore for the classification of colon cancer: a prognostic and accuracy study. Lancet 2018;391:2128-39. [Crossref] [PubMed]
- Chen Y, Meng Z, Zhang L, et al. CD2 Is a Novel Immune-Related Prognostic Biomarker of Invasive Breast Carcinoma That Modulates the Tumor Microenvironment. Front Immunol 2021;12:664845. [Crossref] [PubMed]
- Wu J, Li L, Zhang H, et al. A risk model developed based on tumor microenvironment predicts overall survival and associates with tumor immunity of patients with lung adenocarcinoma. Oncogene 2021;40:4413-24. [Crossref] [PubMed]
- Vanoevelen J, Dode L, Van Baelen K, et al. The secretory pathway Ca2+/Mn2+-ATPase 2 is a Golgi-localized pump with high affinity for Ca2+ ions. J Biol Chem 2005;280:22800-8. [Crossref] [PubMed]
- Martinelli A, Rice ML, Talcott JB, et al. A rare missense variant in the ATP2C2 gene is associated with language impairment and related measures. Hum Mol Genet 2021;30:1160-71. [Crossref] [PubMed]
- Smith AW, Holden KR, Dwivedi A, et al. Deletion of 16q24.1 supports a role for the ATP2C2 gene in specific language impairment. J Child Neurol 2015;30:517-21. [Crossref] [PubMed]
- Jenkins J, Papkovsky DB, Dmitriev RI. The Ca2+/Mn2+-transporting SPCA2 pump is regulated by oxygen and cell density in colon cancer cells. Biochem J 2016;473:2507-18. [Crossref] [PubMed]
- Zhao H, Zhang S, Shao S, et al. Identification of a Prognostic 3-Gene Risk Prediction Model for Thyroid Cancer. Front Endocrinol (Lausanne) 2020;11:510. [Crossref] [PubMed]
- Liu J, Wei Y, Wu Y, et al. ATP2C2 Has Potential to Define Tumor Microenvironment in Breast Cancer. Front Immunol 2021;12:657950. [Crossref] [PubMed]
- Dang DK, Makena MR, Llongueras JP, et al. A Ca(2+)-ATPase Regulates E-cadherin Biogenesis and Epithelial-Mesenchymal Transition in Breast Cancer Cells. Mol Cancer Res 2019;17:1735-47. [Crossref] [PubMed]
- Girault A, Peretti M, Badaoui M, et al. The N and C-termini of SPCA2 regulate differently Kv10.1 function: role in the collagen 1-induced breast cancer cell survival. Am J Cancer Res 2021;11:251-63. [PubMed]
- Paijens ST, Vledder A, de Bruyn M, et al. Tumor-infiltrating lymphocytes in the immunotherapy era. Cell Mol Immunol 2021;18:842-59. [Crossref] [PubMed]
- Lipp JJ, Wang L, Yang H, et al. Functional and molecular characterization of PD1(+) tumor-infiltrating lymphocytes from lung cancer patients. Oncoimmunology 2022;11:2019466. [Crossref] [PubMed]
- Aran D, Hu Z, Butte AJ. xCell: digitally portraying the tissue cellular heterogeneity landscape. Genome Biol 2017;18:220. [Crossref] [PubMed]
- Li T, Fu J, Zeng Z, et al. TIMER2.0 for analysis of tumor-infiltrating immune cells. Nucleic Acids Res 2020;48:W509-14. [Crossref] [PubMed]
- Brummelman J, Mazza EMC, Alvisi G, et al. High-dimensional single cell analysis identifies stem-like cytotoxic CD8(+) T cells infiltrating human tumors. J Exp Med 2018;215:2520-35. [Crossref] [PubMed]
- Borst J, Ahrends T, Bąbała N, et al. CD4(+) T cell help in cancer immunology and immunotherapy. Nat Rev Immunol 2018;18:635-47. [Crossref] [PubMed]
- Oshi M, Asaoka M, Tokumaru Y, et al. CD8 T Cell Score as a Prognostic Biomarker for Triple Negative Breast Cancer. Int J Mol Sci 2020;21:6968. [Crossref] [PubMed]
- Steinman RM. Lasker Basic Medical Research Award. Dendritic cells: versatile controllers of the immune system. Nat Med 2007;13:1155-9. [Crossref] [PubMed]
- Collin M, Bigley V. Human dendritic cell subsets: an update. Immunology 2018;154:3-20. [Crossref] [PubMed]
- Böttcher JP, Bonavita E, Chakravarty P, et al. NK Cells Stimulate Recruitment of cDC1 into the Tumor Microenvironment Promoting Cancer Immune Control. Cell 2018;172:1022-1037.e14. [Crossref] [PubMed]