Two-dimensional correlated spectroscopy distinguishes clear cell renal cell carcinoma from other kidney neoplasms and non-cancer kidney
Introduction
Renal cell carcinoma (RCC) represents a heterogeneous group of cancers with varying clinical outcomes, ranging from indolent to metastatic behaviour. Kidney cancer accounts for approximately 2% of all cancers, and each year more than 400,000 new cases are diagnosed worldwide (1). The incidence of RCC has been increasing globally by approximately 2–3% annually over the last decade in most countries (2). Physiological risk factors for RCC that are likely contribute to the increased incidence are smoking, obesity and hypertension, however epidemiological data have demonstrated that the highest increases in both incidence and mortality rate, in the context of RCC, occur in primary lesions, that is, tumors that are confined anatomically to the kidney and have not spread to local and/or distant structures (3). A means of classifying tumors in the kidney preoperatively would provide significant improvement in patient care (4). Up to 20% of enhancing small kidney masses are benign and may not need surgical management (5). A small proportion demonstrate aggressive pathological features and may progress to incurable metastatic disease.
Current kidney mass evaluation strategies do not provide sufficient diagnostic certainty for patients and clinicians, resulting in high rates of unnecessary intervention. This is evidenced by published contemporary surgical series reporting high rates of resected benign lesions. For example, a report evaluating 1,175 robotic partial nephrectomy procedures for tumors ≤4 cm at five academic USA centers concluded that about 50% of patients could have avoided surgery if reliable preoperative diagnostic strategies were available (6). The lack of preoperative diagnostic tools, and few diagnostic chemical markers or risk-stratification tools, makes decision-making difficult for clinicians (7).
This same challenge existed, and was resolved, for preoperative diagnosis of benign versus malignant ovarian lesions. The translational approach was to first understand the magnetic resonance (MR) visible chemicals in ovarian cells (8,9) and biopsies (10) and correlate these with the gold standard histopathology. This methodology allowed the technology to be successfully translated, with significant hardware improvements, for preoperative and noninvasive diagnosis in vivo (11,12). It now provides gynecological surgeons the opportunity to triage patients and plan surgery (13). Here we take the same approach by attempting to identify pathology-specific metabolic, lipid and carbohydrate markers for RCC subtypes and compare them with benign kidney masses and non-cancer kidney cortex. Others have reported biomarker characteristics of some RCC phenotypes, but mainly the clear cell RCC (ccRCC) phenotype (14,15). Here we show that ccRCC tissue, non-ccRCC tumor tissue, and non-cancer kidney cortical tissue have distinct metabolic characteristics that may be useful in the classification of renal lesions.
The use of routine abdominal imaging in the clinical setting has led to the increased detection of stage 1 tumors (2,16). The American Joint Committee on Cancer tumor-node metastasis (AJCC TNM) classification (17) defines stage 1 as localized to the kidney and ≤7 cm in diameter (T1), with no spread to lymph nodes (N0) or distant organs (M0) (18). The malignant potential of T1 lesions ranges from benign lesions, such as renal oncocytoma, to malignant phenotypic variants such as ccRCC. Currently, T1 lesions are excised surgically, and final diagnosis is made post-operatively using histopathology. Core needle biopsy may play a role in risk stratification of T1 lesions; however, the clinical utility has remained controversial (19,20).
The current study uses two-dimensional COrrelated SpectroscopY (2D L-COSY) to evaluate metabolic profiles from human renal biopsy samples compared with their histopathology. Unlike one-dimensional (1D) spectroscopy, 2D L-COSY offers the advantage of being able to filter overlapping resonances based on their T2 relaxation rate, which allows us to identify individual molecules that resonate at similar frequencies (21,22). We used both multivariate statistical modelling and univariate statistical analysis to evaluate 2D L-COSY data. Using chemical differences recorded among RCC subgroups and the frequencies at which they resonate, it may be possible to translate this method for preoperative in vivo diagnosis in a clinical magnetic resonance imaging (MRI) scanner using the 1D single voxel method and modern pattern recognition techniques (23,24). We present the following article in accordance with the MDAR (25) reporting checklist (available at https://tau.amegroups.com/article/view/10.21037/tau-21-1082/rc).
Methods
Ethical approval
The study was conducted in accordance with the Declaration of Helsinki (as revised in 2013). The study was approved by the University of Queensland Medical Research Ethics Committee (approval number: 20016001215) and the Metro South Health Human Research Ethics Committee (HREC/16/QPAH/353 and HREC/15/QRBQ/610). All elements of this study were carried out in accordance with approved guidelines and regulations from relevant institutional and governance bodies. Informed consent was obtained from all participants in the study.
Study population
The study included 169 tissue samples from 70 patients, undergoing nephrectomy for suspected RCC. There were 19 females with a mean age of 58±14 years and 51 males with a mean age of 58±14 years. Table 1 summarises the demographic characteristics of the patient cohort by gender. The gender distinction was made to demonstrate the distinct higher incidence of ccRCC in males than females, versus the gender differences in non-ccRCC.
Table 1
Demographics | Male | Female |
---|---|---|
ccRCC (total) | 34 | 12 |
AJCC clinical T stage | ||
T1 | 13 | 3 |
T2 | 3 | 1 |
T3 | 17 | 7 |
T4 | 1 | 1 |
ISUP/WHO Grade | ||
1 | 1 | 1 |
2 | 15 | 5 |
3 | 12 | 4 |
4 | 6 | 2 |
Non-ccRCC (total) | 17 | 7 |
Subtype | ||
Oncocytoma | 5 | 3 |
Angiomyolipoma | 2 | |
Chromophobe | 2 | 1 |
Papillary T1 | 3 | |
Papillary T2 | 3 | 1 |
Mucinous tubular and spindle cell carcinoma | 1 | |
Leiomyoma-like | 1 | |
Hybrid oncocytoma/chromophobe | 1 | |
Acquired cystic disease associated RCC | 1 | |
AJCC clinical T stage | ||
T1 | 11 | 4 |
T2 | 2 | 2 |
T3 | 4 | 1 |
T4 | ||
Age group, years | ||
<40 | 2 | |
40–60 | 27 | 11 |
>60 | 22 | 8 |
BMI group | ||
≤24.9 | 12 | 2 |
25–29.9 | 14 | 6 |
≥30 | 25 | 11 |
RCC, renal cell carcinoma; ccRCC, clear cell renal cell carcinoma; AJCC, American Joint Committee on Cancer; ISUP/WHO, International Society of Urologic Pathologists/World Health Organization; BMI, body mass index.
Tissue sampling
Excisional tumor biopsy samples were obtained in the operating theatre, immediately following nephrectomy. Tumor tissue samples were carefully excised from peripheral tumor edges, avoiding areas of central necrosis, and with surgical margins considered. Adjacent non-cancer cortical tissue from radial nephrectomy specimens was excised distally to the tumor, when available. Adjacent non-cancer tissue was not readily available in the setting of partial nephrectomy. Approximately 50 mg of tissue for magnetic resonance spectroscopy (MRS) was placed in a pre-weighed vial containing phosphate buffered saline/deuterium oxide (PBS/D2O), and subsequently snap frozen in liquid nitrogen and stored at −80 ℃. Prior to MRS, tissue samples were quickly thawed, weighed, washed in PBS/D2O, and placed in a sterile 5 mm nuclear MR (NMR) tube containing PBS/D2O, as per previous ex-vivo biopsy MRS sample preparation protocols (26). Tissue samples were also formalin-fixed for routine histopathology using Queensland Health Pathology Services.
MRS
1D and 2D L-COSY spectra were acquired (27) using an Avance Neo 400 NMR Spectrometer (Bruker Biospin GmbH, Karlsrhule, Germany) operating with Topspin 4.0.5. Spectra were recorded at 37 ℃ with residual water suppressed by selective gated irradiation. 1D spectra were acquired over a spectral width of 3,968 Hz (9.92 ppm) with a 90° pulse of 10 µs, an acquisition time of 4.13 s, 32,768 data points, 2 dummy scans and 32 accumulations, a total relaxation delay of 2.00 s, over a time of 3 min 29 s. For 2D L-COSY, 1,024 complex data pairs were acquired in the T2 time domain over a spectral width (SW2) of 3,968 Hz (9.92 ppm). Data sampling in T1 was acquired with an initial delay between the two 90° pulses, tl min =1 ms, an increment time of 252 µs, and a relaxation delay of 1.00 s. The number of tl increments was 256, each of 4 transients, over a time of 39 min 42 s.
Post acquisitional processing
Standard data processing techniques were applied, including Fourier transformation, phase correction, baseline correction and calibration (28,29). For 1D MRS, spectra were processed using Topspin software (Version 4.0.5, Bruker Biospin GmbH, Karlsrhule, Germany), a widely used data processing tool. The resulting output data were visualized using Mnova 14.1.2 (Mestrelab Research). For 2D L-COSY, raw spectral data were post-processed with standard processing parameters using Mnova 14.1.2. Signal processing, using a Lorenzian broadening-Gaussian broadening (LB-GB) transformation (27) of LB =−50.00 Hz, GB =0.14 (equivalent to 25 Hz) across both the T2 domain and T1 domain of the spectra, was used to optimize the visibility of amino acid and triglyceride molecules and reduce noise levels across the spectra, similar to work by Delikatny et al. (27). Spectra were calibrated on the chemical shift of choline at F1, F2: 3.23, 3.23 ppm. Following spectral processing and calibration, all signals of interest were identified, and peaks were selected across the spectrum to create a template for integration. Peaks were selected and centred manually to ensure that a maximal signal of interest was captured while minimizing signals from noise. The template was then saved and applied to the spectrum of each sample. Absolute integration values were then calculated in Mnova 14.1.2. Using a cut-off signal-to-noise ratio of >5, 134 cross-peaks were identified, with peak and cross-peak integrals collated into a data matrix for subsequent analysis. Absolute integration buckets were normalized to the integral of the creatine peak at the diagonal (F1, F2: 3.03, 3.03 ppm) (29,30).
Histopathology
The kidney samples were fixed in 10% buffered formalin for 24–48 h, then transferred to PBS, embedded routinely in paraffin and sectioned onto glass histology slides at 5 µm thickness. For hematoxylin and eosin (H&E) staining, sections on slides were deparaffinized, rehydrated through alcohols to water, stained with Mayer’s hematoxylin for 5 min, washed in water then blued with sodium bicarbonate (1 g in 1 L water) to clearly distinguish nuclei, dehydrated to 90% ethanol, stained with Eosin Y for 2 min, washed with 100% ethanol, then xylene, and then mounted with glass coverslips using Depex mounting medium. Slides were scanned using an Olympus VS120 Slidescanner Microscope at ×40 magnification using VS-ASW software. The digital images were then visualised using Olympus OlyVIA 3.2.1 software. Histopathological diagnosis was determined by the Princess Alexandra Hospital’s pathology service using H&E-stained sections and validated by an expert urological pathologist (HS). The World Health Organisation/International Society of Urologic Pathologists (ISUP/WHO) grading system for RCC was used to stage, grade and classify histological subtypes (31).
Training and validation cohorts
Prior to multivariate statistical analysis, the training and validation cohorts were determined using a class-balancing algorithm that determined an approximate 75:25 training: validation split, using the sample function in R (R: A Language and Environment for Statistical Computing). This resulted in a training cohort of 126 samples and a validation cohort of 43 samples.
Statistical analysis
Multivariate statistical analysis and modelling were undertaken using the SIMCA-16 software package (Umetrics, Sweden). Data buckets were normalized to the integral of the creatine peak on the diagonal (F1, F2: 3.03, 3.03 ppm) (29,30) and univariate scaled. Principal component analysis (PCA), a data projection method that allows simplified data visualization of large datasets, was applied in the first instance. PCA of the training cohort, including 2D L-COSY data for 126 biopsy samples, was used to determine trends and clusters based on clinical data (histological subtype, gender, age, and body mass index groups). Trends observed in PCA were further analyzed using orthogonal projection to latent structures with discriminant analysis (OPLS-DA) to reveal the metabolic classifiers contributing to group separation.
Results
Spectra from 2D L-COSY differentiate cancer subtypes and non-cancer kidney
Shown in Figures 1,2 are examples of typical spectra from 1D MRS and 2D L-COSY for the histological subtypes seen in Figure 3 (non-cancer kidney, two non-ccRCC as oncocytoma and papillary Type 1 RCC, and ccRCC). In Figure 1, high quality 1D spectra are demonstrated as a comparison with the more informative 2D L-COSY spectra. 1D spectra are recorded on a frequency axis to demonstrate the chemical shift in representative samples on non-cancer kidney cortex, benign oncocytoma, papillary type-1 RCC, and ccRCC. In Figure 2 of 2D L-COSY spectra of non-cancer kidney cortex, benign oncocytoma, papillary type-1 RCC, and ccRCC, resonance assignments of major lipid and cholesterol moieties are labelled. A visible increase is recorded in this series. Resonance assignments were made based on previous reports (30,32,33) as well as using the Human Metabolome Database (34).
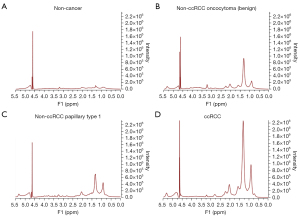
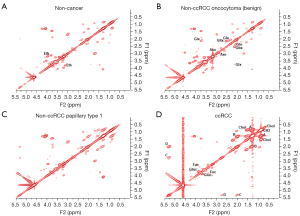
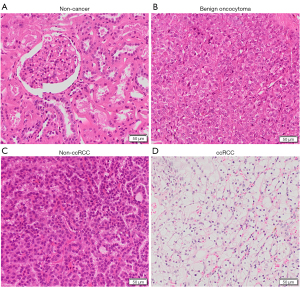
Results of multivariate statistical analysis
Using PCA, data from the training set consisting of 126 spectra were calculated and PCA of all training samples are shown in Figure 4. Scores plots demonstrated clustering according to histological subtype, with ccRCC tissues clustering away from non-cancer kidney and non-ccRCC tissues, but some overlap of non-cancer kidney and non-ccRCC tissues (Figure 4A). All data were subsequently modelled into supervised OPLS-DA and validated by 300-fold permutation analysis (Figure 4B). Overall, OPLS-DA models revealed excellent separation based on tissue subtype. OPLS-DA is a supervised technique that builds off trends observed in PCA and models these data into the pre-assigned classes. The classes used to build the OPLS model were based on the clustering observed in PCA (non-cancer kidney cortex, non-ccRCC and ccRCC tissues). OPLS-DA models were successful in classifying the three tissue types using all 134 spectroscopic features, (R2Y =0.70, Q2 =0.60). Two outliers of the non-ccRCC group were seen and identified as benign angiomyolipomas.
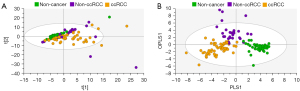
In Figure 5, exclusion of the non-cancer control samples from subsequent PCA modelling provided unsupervised clustering of ccRCC and non-ccRCC samples (Figure 5A). In Figure 5B, OPLS-DA plots demonstrate clear separation of subtypes using supervised classification of tissues by group. The model was also able to discriminate between ccRCC and non-ccRCC for the smaller T1 lesion samples analyzed in this study with a sensitivity of 97.3%. These differences are demonstrated in Figure 6, which also shows distribution of non-cancer tissue. However, no clear distinction was found when “all RCC” were compared with benign lesions (data not shown).
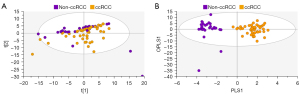
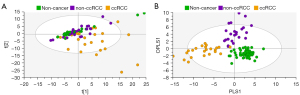
Using the OPLS-DA model from Figure 4B, the ‘Predict’ function on SIMCA software was utilized to validate the model on an additional cohort of 43 biopsy samples. This validation achieved an overall sensitivity of 86% (Table 2). Of the five misclassified samples, three were ccRCC tissues that, on re-review, were found to be misclassified as non-ccRCC, and two non-ccRCC tissues that were misclassified as non-cancer, or ccRCC, respectively. All non-cancer kidney samples were predicted correctly. Using the OPLS-DA model which excluded the non-cancer kidney samples (Figure 5B), the validation cohort of 27 samples distinguished all non-ccRCC from ccRCC tissue with an overall sensitivity of 92.6%, with one non-ccRCC and one ccRCC being misclassified. Wilson-Brown statistical analysis of this validation cohort gave a positive predictive value of 0.9 (90%) and a negative predictive value of 0.94 (94%) for ccRCC.
Table 2
OPLS-DA model | Number | R2Y | Q2 | Model sensitivity |
---|---|---|---|---|
Training cohort: non-cancer vs. non-ccRCC vs. ccRCC | 126 | 0.708 | 0.608 | 96.8% (122/126) |
Validation cohort: non-cancer vs. non-ccRCC vs. ccRCC | 43 | – | – | 86% (37/43) |
Training cohort: non-ccRCC vs. ccRCC | 79 | 0.965 | 0.743 | 100% (79/79) |
Validation cohort: non-ccRCC vs. ccRCC | 27 | – | – | 92.6% (25/27) |
OPLS-DA, orthogonal projection to latent structure with discriminant analysis; non-cancer, non-cancer kidney tissue from nephrectomised kidney distant from cancer; ccRCC, clear cell renal cell carcinoma.
Results of univariate statistical analysis
Resonance assignments are listed in Table 3. The resonances from multivariate analysis with Variable Importance for the Projection (VIP) >1.2 were selected for univariate analysis, as these had the highest contribution to group separation. The resulting 26 resonances of interest were univariate scaled, and log2 transformed using Graphpad Prism (Version 9). Chemical makers are ordered by the F2 parts per million (PPM) co-ordinates, highest to lowest. Using unpaired t-tests, integration volumes were compared across tissue types, with a P<0.05 considered to be significant.
Table 3
F2 PPM | F1 PPM | Resonance assignment | ccRCC cf non-cancer | P value | Non-ccRCC cf non-cancer | P value | ccRCC cf non-ccRCC | P value |
---|---|---|---|---|---|---|---|---|
5.30 | 2.73 | Lipid D | ↑ | <0.0001 | ↑ | <0.0004 | ↑ | <0.0001 |
3.88 | 3.71 | D-glucose peak3 | ↑ | <0.0001 | ns | 0.0368 | ↑ | <0.0001 |
3.81 | 3.40 | D-glucose peak2 | ↑ | <0.0005 | ns | 0.0524 | ↑ | <0.0005 |
3.80 | 3.13 | Ethanolamine Peak1 | ↓ | <0.0001 | ↓ | <0.0001 | ns | 0.9956 |
3.76 | 2.14 | Glutamine/Glutamate Peak1 | ↑ | <0.0001 | ↑ | <0.0001 | ns | 0.1007 |
3.72 | 2.05 | Glutamate peak1 | ↑ | <0.0005 | ↑ | <0.0025 | ns | 0.3656 |
3.70 | 3.87 | D-glucose peak3' | ↑ | <0.0001 | ns | 0.8642 | ↑ | <0.0001 |
3.69 | 3.40 | Fucose peak2 | ↑ | <0.0001 | ns | 0.0651 | ↑ | <0.0001 |
3.42 | 3.42 | Fucose/D-glucose | ↑ | <0.0001 | ns | 0.7066 | ↑ | <0.0002 |
3.41 | 3.71 | Fucose peak2' | ↑ | <0.0001 | ns | 0.9304 | ↑ | <0.0001 |
3.38 | 3.81 | D-glucose peak2' | ↑ | <0.0001 | ns | 0.0658 | ↑ | <0.0001 |
2.84 | 2.95 | L-asparagine peak1' | ↑ | <0.0001 | ↑ | <0.0001 | ns | 0.5649 |
2.77 | 2.77 | α-ketobutyrate* | ↑ | <0.0001 | ↑ | <0.0002 | ↑ | <0.0001 |
2.65 | 2.79 | Aspartate peak1' | ↑ | <0.0001 | ns | 0.3926 | ↑ | <0.0001 |
2.54 | 2.14 | Glutathione peak1 | ↑ | <0.0001 | ↑ | <0.0001 | ↓ | <0.0001 |
2.45 | 2.12 | Glutamine peak1 | ↑ | <0.0001 | ↑ | <0.0001 | ns | 0.2924 |
2.14 | 2.54 | Glutathione peak1' | ↑ | <0.0001 | ↑ | <0.0001 | ↓ | <0.0001 |
2.12 | 3.76 | Glutamine/glutamate peak1' | ↑ | <0.0004 | ↑ | <0.0001 | ns | 0.1632 |
2.11 | 2.44 | Glutamine peak1' | ↑ | <0.0001 | ↑ | <0.0001 | ↓ | <0.02 |
2.05 | 0.95 | Isovalerylglycine peak1** | ↑ | <0.0001 | ↑ | <0.0006 | ↑ | <0.0006 |
1.71 | 0.94 | L-leucine peak1 | ↑ | <0.0001 | ns | 0.1695 | ↑ | <0.0016 |
1.52 | 0.86 | Cholesterol peak2 | ↑ | <0.0001 | ↑ | <0.0001 | ↑ | <0.0001 |
0.95 | 2.05 | Isovalerylglycine peak1'** | ↑ | <0.0001 | ↑ | <0.0001 | ↑ | <0.0006 |
0.91 | 0.91 | Lipid CH3 | ↑ | <0.0001 | ↑ | <0.0001 | ↑ | <0.0001 |
0.87 | 1.51 | Cholesterol peak2' | ↑ | <0.0001 | ↑ | <0.0001 | ↑ | <0.0001 |
0.70 | 0.70 | Cholesterol peak1 | ↑ | <0.0001 | ↑ | <0.0001 | ↑ | <0.0001 |
Chemical markers are ordered by the F2 PPM co-ordinates, highest to lowest. ↑, increased; ↓, decreased; ns, non-significant; ', resonance cross-peak above the diagonal in 2D-COSY. *, 2.77 on the spectral diagonal is considered a composite peak of α-ketobutyrate or its derivatives; **, by-product of leucine metabolism, most probably isovalerylglycine. F1 and F2 PPM, frequency domains in PPM; ccRCC, clear cell renal cell carcinoma; non-ccRCC, non-clear cell RCC; non-cancer, non-cancer kidney tissue from nephrectomised kidney distant from cancer; cf, compared with; PPM, parts per million.
Discussion
There is an urgent need for pre-operative risk stratification of small kidney masses. Traditional imaging methods lack the specificity to distinguish aggressive versus indolent tumor subtypes in localized kidney masses, which often results in surgical overtreatment. International urological guidelines are starting to consider active surveillance in the management of clinically localized RCC in patients that are amenable and have undergone a careful risk-assessment (35). Developing a tool that could provide non-invasive classification would allow patients and clinicians to make better informed decisions about the pursuit of active surveillance for small renal masses. This study demonstrates that chemical markers can separate distinct RCC pathologies. The capacity to distinguish between these pathological classes could greatly benefit the management of RCC in both the selection of targeted therapies, and other treatment options including active surveillance. Here we show that chemical features, identified using 2D L-COSY, can provide useful information that replaces or augments histological classification of kidney mass subtypes, and have potential for translational applications. The general increase in use of abdominal imaging techniques, such as computed tomography (CT) scans, contributes to increased detection of kidney masses. Another concern, unrelated to incidence rates, is that most early-stage kidney cancers are clinically silent, and only show the classic triad of patient symptoms of hematuria, flank pain and palpable abdominal mass at a later stage of disease progression.
This raises a significant question about the optimal clinical management of patients presenting with localized kidney masses. Given the increasing incidence and mortality rates, it is imperative for clinicians to select patients who will benefit from active treatment and spare those patients for whom treatment would likely be unnecessary. In making this decision, the histopathological classification of RCC is extremely important for both the prognosis and management of the disease. Of RCC subtypes, ccRCC is the most frequently occurring kidney cancer, accounting for up to 80% of kidney lesions (36). This subtype is also associated with poorer prognosis, compared with most other subtypes of RCC (37). Relatively common non-ccRCC phenotypic variants include papillary RCC and chromophobe RCC. Benign renal oncocytoma is often considered alongside the non-ccRCC. The heterogeneity and knowledge of RCC tumor subtypes continues to expand, as new subtypes continue to be described (31).
Despite the increase in the incidence of RCC, the management of localized RCC has largely remained unchanged, with nephrectomy being standard of care (38). Percutaneous core biopsy is rarely used to diagnose the kidney masses detected, mainly because of the heterogeneity of RCC and the chance for misdiagnosis with histopathology, even when several of the small core biopsies are considered. Thus, developments in the field of diagnostic imaging and metabolomics, such as through MRS, may provide helpful information about the characterisation and aggressiveness of RCC. Currently, even diagnostic imaging fails to make this distinction, with a proportion of T1 lesions being upstaged to T3, due to anatomical pathological invasion that is only identifiable on the histological level.
MR-based chemical evaluation has shown promising results in classifying RCC in small cohort studies (39). Kidney cancer has been described as a disease of aberrant cellular metabolism (40), the by-products of which may be exploited as potential diagnostic markers. Previous studies investigating metabolism in RCC have identified the importance of aberrant lipid (41), and glucose, glutathione, and amino acid changes (42) that are likely to be key drivers in the pathogenesis of RCC. Adipogenesis is a known hallmark of ccRCC (41). Our data demonstrate an overall increase in lipid and cholesterol chemical signatures in ccRCC, compared with both non-ccRCC and non-cancer kidney tissue, indicating that lipid and cholesterol changes may have a significant role in translational diagnostic applications for ccRCC. Glucose and glycogen have been reported to alter in different cancer types and their different developmental stages (14,42,43). In our study, we see an increase in intracellular glucose moieties in ccRCC but not in non-ccRCC, which may reflect unique energy requirements in the ccRCC which are, generally, more rapidly proliferating.
Amino acid metabolism is also reported to have a key role in biomarker aberrations seen in ccRCC tumors; many other studies have also identified amino acid alterations in cancer (14,44,45). In the current study, we observed different levels of glutamine, glutamate, leucine, asparagine and aspartate between our tumor and non-cancer groups. Branched chain amino acids (BCAA) uptake and BCAA pathways are activated in many tumors (46). They are used for protein synthesis, in the production of glutamate and glutamine and in downstream energy production via the tricarboxylic acid cycle (44,45,47). In this study, we observed a significant increase in the BCAA leucine between non-cancer and ccRCC, and an increase in the leucine catabolite, isovalerylglycine, and the threonine and methionine catabolite, α-ketobutyrate between non-cancer and all tumor groups. The chemical differences for isovalerylglycine and α-ketobutyrate were higher, 55% and 108% respectively, in ccRCC than the non-ccRCC and non-cancer kidney.
In another study, ccRCC tissue was reported to have higher glutamine when compared to adjacent non-cancer kidney (14). There have, however, been few studies examining the role of glutamine in non-ccRCC tissues. Our results indicate that glutamine is significantly increased in ccRCC tissues and non-ccRCC tissues, when compared with adjacent non-cancer kidney. The glutamine pathway is an essential mechanism of cellular anabolism and tumor cell growth and has been implicated as a cellular adaptation in the attenuation of oxidative stress (47). Glutathione is an abundant antioxidant and a redox regulator that has been studied extensively in cancer cell metabolism. Glutathione is also a potent neutralizer of reactive oxygen species, a cofactor for enzymatic reactions and a regulator of cellular proliferation and apoptosis. Relating to RCC, glutathione metabolism has been identified as a marker of aggressiveness and correlates with poor prognostic outcome (42,45). In this study, we observed an increase in glutathione in ccRCC, and even more so in non-ccRCC, compared to non-cancer kidney tissue.
Fucose and fucosylation are associated with cancer cell growth, metastasis and invasion, with fucosidase being down regulated in a number of cancer types, and agents that target fucosylation having an inhibitory effect on metastasis (48). In the current study, increased fucose levels were differential for ccRCC compared to other tissue types. Finally, several studies have reported increased choline levels associated with tumorigenesis (49,50), however, in our study, choline levels did not contribute significantly to group separation, with a broad standard deviation in both ccRCC and non-ccRCC.
The strength of the current investigation is that it demonstrates distinct chemical characteristics among histological tissue types of kidney masses, and clearly separates ccRCC, which has a poor clinical prognosis, from non-ccRCC and non-cancer kidney with 86% predictive accuracy. This was accomplished with use of multivariate data analysis software and application of experience gained by our team using a similar analysis of ovarian cancer. A limitation of this study was the small number of non-ccRCC available to us, simply because of the low incidence of these subtypes compared with ccRCC. Thus, the non-ccRCC group was heterogeneous, consisting of several histological subtypes and, consequently, we lacked the statistical power to separate distinct histological subtypes among non-ccRCC tumors. To counter this limitation, a much larger patient cohort is needed.
This pre-clinical study utilized excisional tumor tissue biopsies to obtain 2D L-COSY MRS data. We determined that approximately 50 mg of tissue were required to obtain adequate signal from MRS. Therefore, we hypothesize that this technique would not likely be suitable for core needle biopsy samples, which sample significantly less tissue. The aim of this pre-clinical study was to determine if there were differences between histological tumor types that could be visualized using MRS. We have shown that there are observable differences between ccRCC and non-ccRCC tissues. This study was not able to observe differences between RCC and benign lesions (for example, oncocytoma, angiomyolipoma), largely due to a small sample size of benign lesions. Future studies will aim to validate these differences in larger cohorts that include more participants with benign histology. Ultimately, this work is a step towards the ability to translate these findings in vivo, with a hope to improve the diagnosis and management of RCC.
Conclusions
This study provides metabolic insights that could have future diagnostic and/or clinical value. The results of this work demonstrate a clear separation between ccRCC and non-ccRCC and non-cancer kidney cortical tissue from tumor-bearing patients. Key chemical signatures were responsible for subtype separation, with classifying chemical markers for ccRCC being cholesterol, lipid moieties, glucose and fucose. Glutamine and glutathione were increased in tumor tissues (ccRCC and even more so in non-ccRCC), indicating that glutamatergic metabolism may provide diagnostic insights in the separation of these histopathological entities. Additional analysis that would optimize and validate the data analytical technique with respect to comparisons of ccRCC from non-ccRCC would be useful, specifically focusing on a clinical cohort of patients with localized (primary) kidney masses. The development of a 1D MRS protocol using an in vivo MR imaging clinical scanner is the next step in the translational application of this work.
Acknowledgments
Funding: This research was supported by a Project Grant (GNT1147077) from the National Health and Medical Research Council of Australia. The research was also supported by a Tour de Cure Research Grant (Australia) and a Metro South Hospital and Health Service Research Support Scheme (Princess Alexandra Hospital SERTA Grant).
Footnote
Reporting Checklist: The authors have completed the MDAR reporting checklist. Available at https://tau.amegroups.com/article/view/10.21037/tau-21-1082/rc
Data Sharing Statement: Available at https://tau.amegroups.com/article/view/10.21037/tau-21-1082/dss
Conflicts of Interest: All authors have completed the ICMJE uniform disclosure form (available at https://tau.amegroups.com/article/view/10.21037/tau-21-1082/coif). SJDV received a University of Queensland Research Training Program Scholarship for her PhD studies and a Randal Silcock Bursary for conference travel. CEM has received research grants from Advance Queensland Research, was a consultant for the National Imaging Facility, and is a shareholder for DatChem Pty Ltd. and Goolwa Pty Ltd. The other authors have no conflicts of interest to declare.
Ethical Statement: The authors are accountable for all aspects of the work in ensuring that questions related to the accuracy or integrity of any part of the work are appropriately investigated and resolved. The study was conducted in accordance with the Declaration of Helsinki (as revised in 2013). The study was approved by the University of Queensland Medical Research Ethics Committee (approval number: 20016001215) and the Metro South Health Human Research Ethics Committee (HREC/16/QPAH/353 and HREC/15/QRBQ/610). All elements of this study were carried out in accordance with approved guidelines and regulations from relevant institutional and governance bodies. Informed consent was obtained from all participants in the study.
Open Access Statement: This is an Open Access article distributed in accordance with the Creative Commons Attribution-NonCommercial-NoDerivs 4.0 International License (CC BY-NC-ND 4.0), which permits the non-commercial replication and distribution of the article with the strict proviso that no changes or edits are made and the original work is properly cited (including links to both the formal publication through the relevant DOI and the license). See: https://creativecommons.org/licenses/by-nc-nd/4.0/.
References
- Bray F, Ferlay J, Soerjomataram I, et al. Global cancer statistics 2018: GLOBOCAN estimates of incidence and mortality worldwide for 36 cancers in 185 countries. CA Cancer J Clin 2018;68:394-424. [Crossref] [PubMed]
- Znaor A, Lortet-Tieulent J, Laversanne M, et al. International variations and trends in renal cell carcinoma incidence and mortality. Eur Urol 2015;67:519-30. [Crossref] [PubMed]
- Turner RM 2nd, Morgan TM, Jacobs BL. Epidemiology of the Small Renal Mass and the Treatment Disconnect Phenomenon. Urol Clin North Am 2017;44:147-54. [Crossref] [PubMed]
- Giménez-Bachs JM, Salinas-Sánchez AS. Improving the diagnosis of renal masses: can we approach the histological diagnosis to the image? Ann Transl Med 2019;7:56. [Crossref] [PubMed]
- Tomaszewski JJ, Uzzo RG, Smaldone MC. Heterogeneity and renal mass biopsy: a review of its role and reliability. Cancer Biol Med 2014;11:162-72. [PubMed]
- Rahbar H, Bhayani S, Stifelman M, et al. Evaluation of renal mass biopsy risk stratification algorithm for robotic partial nephrectomy--could a biopsy have guided management? J Urol 2014;192:1337-42. [Crossref] [PubMed]
- Tsivian M, Mouraviev V, Albala DM, et al. Clinical predictors of renal mass pathological features. BJU Int 2011;107:735-40. [Crossref] [PubMed]
- Mackinnon WB, May GL, Mountford CE. Esterified cholesterol and triglyceride are present in plasma membranes of Chinese hamster ovary cells. Eur J Biochem 1992;205:827-39. [Crossref] [PubMed]
- Mackinnon WB, Dyne M, Hancock R, et al. Malignancy-related characteristics of wild type and drug-resistant Chinese hamster ovary cells. Pathology 1993;25:268-76. [Crossref] [PubMed]
- Mountford C, Smith I, Bourne R. Correlation of Histopathology with Magnetic Resonance Spectroscopy of Human Biopsies. In Graham Webb (Ed.), Modern Magnetic Resonance, London: Springer; 2006:33-8.
- Heintz A, Odicino F, Maisonneuve P, et al. Carcinoma of the Ovary. Int J Gynaecol Obstet 2006;95:S161-92. [Crossref]
- Mountford C, Stanwell P, Ferrier A, et al. In vivo spectroscopy and imaging of the ovary in vivo at 3 tesla and spectroscopy on biopsy at 8.5 tesla. Journal of Women's Imaging 2005;7:71-6. [Crossref]
- Stanwell P, Russell P, Carter J, et al. Evaluation of ovarian tumors by proton magnetic resonance spectroscopy at three Tesla. Invest Radiol 2008;43:745-51. [Crossref] [PubMed]
- Hakimi AA, Reznik E, Lee CH, et al. An Integrated Metabolic Atlas of Clear Cell Renal Cell Carcinoma. Cancer Cell 2016;29:104-16. [Crossref] [PubMed]
- Catchpole G, Platzer A, Weikert C, et al. Metabolic profiling reveals key metabolic features of renal cell carcinoma. J Cell Mol Med 2011;15:109-18. [Crossref] [PubMed]
- Hollingsworth JM, Miller DC, Daignault S, et al. Rising incidence of small renal masses: a need to reassess treatment effect. J Natl Cancer Inst 2006;98:1331-4. [Crossref] [PubMed]
- Paner GP, Stadler WM, Hansel DE, et al. Updates in the Eighth Edition of the Tumor-Node-Metastasis Staging Classification for Urologic Cancers. Eur Urol 2018;73:560-9.
- AJCC Cancer Staging Manual. 8th ed. New York, NY: Springer; 2017.
- Pandharipande PV, Gervais DA, Hartman RI, et al. Renal mass biopsy to guide treatment decisions for small incidental renal tumors: a cost-effectiveness analysis. Radiology 2010;256:836-46. [Crossref] [PubMed]
- Sanchez A, Feldman AS, Hakimi AA. Current Management of Small Renal Masses, Including Patient Selection, Renal Tumor Biopsy, Active Surveillance, and Thermal Ablation. J Clin Oncol 2018;36:3591-600. [Crossref] [PubMed]
- Williams PG, Saunders JK, Dyne M, et al. Application of a T2-filtered COSY experiment to identify the origin of slowly relaxing species in normal and malignant tissue. Magn Reson Med 1988;7:463-71. [Crossref] [PubMed]
- Quadrelli S, Ribbons K, Arm J, et al. 2D in-vivo L-COSY spectroscopy identifies neurometabolite alterations in treated multiple sclerosis. Ther Adv Neurol Disord 2019;12:1756286419877081. [Crossref] [PubMed]
- Stanwell P, Siddall P, Keshava N, et al. Neuro magnetic resonance spectroscopy using wavelet decomposition and statistical testing identifies biochemical changes in people with spinal cord injury and pain. Neuroimage 2010;53:544-52. [Crossref] [PubMed]
- Siddall PJ, Stanwell P, Woodhouse A, et al. Magnetic resonance spectroscopy detects biochemical changes in the brain associated with chronic low back pain: a preliminary report. Anesth Analg 2006;102:1164-8. [Crossref] [PubMed]
- Macleod M, Collings AM, Graf C, et al. The MDAR (Materials Design Analysis Reporting) Framework for transparent reporting in the life sciences. Proc Natl Acad Sci U S A 2021;118:e2103238118. [Crossref] [PubMed]
- Swindle P, McCredie S, Russell P, et al. Pathologic characterization of human prostate tissue with proton MR spectroscopy. Radiology 2003;228:144-51. [Crossref] [PubMed]
- Delikatny EJ, Hull WE, Mountford CE. The effect of altering time domains and window functions in two-dimensional proton COSY spectra of biological specimens. Journal of Magnetic Resonance 1969;1991:563-73.
- Roberts MJ, Schirra HJ, Lavin MF, et al. NMR-based metabolomics: global analysis of metabolites to address problems in prostate cancer. Cervical, Breast and Prostate Cancer. Edited by iConcept Press. Tokwawan, Kowloon, Hong Kong: iConcept Press. 2014:1-43.
- Ramadan S, Andronesi O, Stanwell P, et al. In Vivo Two Dimensional MR Spectroscopy Compares the Biochemistry of the Human Brain and Glioblastoma. Radiology 2011;259:540-9. [Crossref] [PubMed]
- Govindaraju V, Young K, Maudsley AA. Proton NMR chemical shifts and coupling constants for brain metabolites. NMR Biomed 2000;13:129-53. [Crossref] [PubMed]
- Samaratunga H, Gianduzzo T, Delahunt B. The ISUP system of staging, grading and classification of renal cell neoplasia. J Kidney Cancer VHL 2014;1:26-39. [Crossref] [PubMed]
- Mountford C, Lean C, Malycha P, et al. Proton spectroscopy provides accurate pathology on biopsy and in vivo. J Magn Reson Imaging 2006;24:459-77. [Crossref] [PubMed]
- Mountford CE, Doran S, Lean CL, et al. Proton MRS can determine the pathology of human cancers with a high level of accuracy. Chem Rev 2004;104:3677-704. [Crossref] [PubMed]
- Wishart DS, Feunang YD, Marcu A, et al. HMDB 4.0: the human metabolome database for 2018. Nucleic Acids Res 2018;46:D608-17. [Crossref] [PubMed]
- Campbell SC, Uzzo RG, Karam JA, et al. Renal Mass and Localized Renal Cancer: Evaluation, Management, and Follow-up: AUA Guideline: Part II. J Urol 2021;206:209-18. [Crossref] [PubMed]
- Rini BI, Campbell SC, Escudier B. Renal cell carcinoma. Lancet 2009;373:1119-32. [Crossref] [PubMed]
- Cheville JC, Lohse CM, Zincke H, et al. Comparisons of outcome and prognostic features among histologic subtypes of renal cell carcinoma. Am J Surg Pathol 2003;27:612-24. [Crossref] [PubMed]
- Ljungberg B, Bensalah K, Canfield S, et al. EAU guidelines on renal cell carcinoma: 2014 update. Eur Urol 2015;67:913-24. [Crossref] [PubMed]
- Katz-Brull R, Rofsky NM, Morrin MM, et al. Decreases in free cholesterol and fatty acid unsaturation in renal cell carcinoma demonstrated by breath-hold magnetic resonance spectroscopy. Am J Physiol Renal Physiol 2005;288:F637-41. [Crossref] [PubMed]
- Ishikawa I, Honda R, Yamada Y, et al. Renal cell carcinoma detected by screening shows better patient survival than that detected following symptoms in dialysis patients. Ther Apher Dial 2004;8:468-73. [Crossref] [PubMed]
- Gebhard RL, Clayman RV, Prigge WF, et al. Abnormal cholesterol metabolism in renal clear cell carcinoma. J Lipid Res 1987;28:1177-84. [Crossref] [PubMed]
- DiNatale RG, Sanchez A, Hakimi AA, et al. Metabolomics informs common patterns of molecular dysfunction across histologies of renal cell carcinoma. Urol Oncol 2020;38:755-62. [Crossref] [PubMed]
- Wettersten HI. Reprogramming of Metabolism in Kidney Cancer. Semin Nephrol 2020;40:2-13. [Crossref] [PubMed]
- Gao H, Dong B, Jia J, et al. Application of ex vivo (1)H NMR metabonomics to the characterization and possible detection of renal cell carcinoma metastases. J Cancer Res Clin Oncol 2012;138:753-61. [Crossref] [PubMed]
- Pandey N, Lanke V, Vinod PK. Network-based metabolic characterization of renal cell carcinoma. Sci Rep 2020;10:5955. [Crossref] [PubMed]
- Ananieva EA, Wilkinson AC. Branched-chain amino acid metabolism in cancer. Curr Opin Clin Nutr Metab Care 2018;21:64-70. [Crossref] [PubMed]
- Wettersten HI, Hakimi AA, Morin D, et al. Grade-Dependent Metabolic Reprogramming in Kidney Cancer Revealed by Combined Proteomics and Metabolomics Analysis. Cancer Res 2015;75:2541-52. [Crossref] [PubMed]
- Keeley TS, Yang S, Lau E. The Diverse Contributions of Fucose Linkages in Cancer. Cancers (Basel) 2019;11:1241. [Crossref] [PubMed]
- Ackerstaff E, Glunde K, Bhujwalla ZM. Choline phospholipid metabolism: a target in cancer cells? J Cell Biochem 2003;90:525-33. [Crossref] [PubMed]
- Wen S, He Y, Wang L, et al. Aberrant activation of super enhancer and choline metabolism drive antiandrogen therapy resistance in prostate cancer. Oncogene 2020;39:6556-71. [Crossref] [PubMed]