A Review on Vibration Signal Analysis Techniques Used for Detection of Rolling Element Bearing Defects
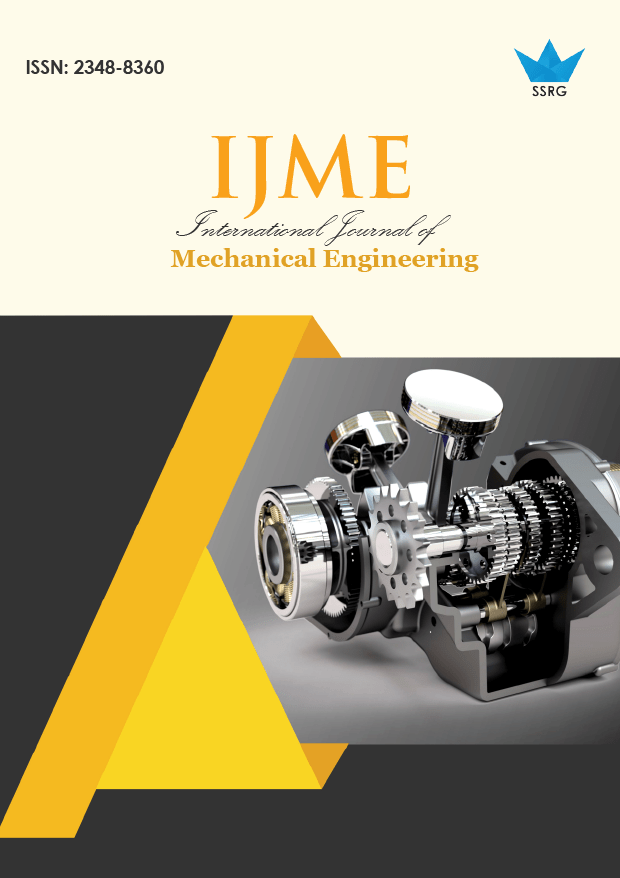
International Journal of Mechanical Engineering |
© 2021 by SSRG - IJME Journal |
Volume 8 Issue 1 |
Year of Publication : 2021 |
Authors : Prashant H. Jain, Dr. Santosh P. Bhosle |
How to Cite?
Prashant H. Jain, Dr. Santosh P. Bhosle, "A Review on Vibration Signal Analysis Techniques Used for Detection of Rolling Element Bearing Defects," SSRG International Journal of Mechanical Engineering, vol. 8, no. 1, pp. 14-29, 2021. Crossref, https://doi.org/10.14445/23488360/IJME-V8I1P103
Abstract:
Almost all machines having rotating parts contain rolling element bearings to support the rotating parts during power transmission. Bearing failure is a major cause of the breakdown of machines. Hence it is necessary to identify the defects and their severity in their early stage to avoid breakdown of the machine and catastrophic damages. Defective bearings generation vibrations and various vibration signal analysis techniques have been developed by researchers for bearing condition monitoring. This paper presents an introduction and updated review of vibration signal analysis techniques used for the detection of defects in rolling element bearings. In this paper, vibration signal analysis techniques used for bearing defect detection are reviewed according to their classification viz. time domain, frequency domain, and time-frequency domain. This study will help the researchers to understand recent developments in the detection of defects of bearings from their vibration signals.
Keywords:
Vibration signal analysis techniques, bearing defects, time-domain, frequency-domain, time-frequency domain
References:
[1] N. Tandon and A. Choudhury, An Analytical Model for the Prediction of the Vibration Response of Rolling Element Bearings due to a Localized Defect, Journal of Sound and Vibration, 205(3) (1997) 275–292.
[2] S. Singh, C. Q. Howard and C. H. Hansen, An extensive review of vibration modeling of rolling element bearings with localized and extended defects, Journal of Sound and Vibration, 357(2015) 300–330.
[3] I. El-Thalji and E. Jantunen, A summary of fault modeling and predictive health monitoring of rolling element bearings, Mechanical Systems & Signal Process, 60–61 (2015) 252–272.
[4] A. Sharma, N. Upadhyay, P. K. Kankar, and M. Amarnath, Nonlinear dynamic investigations on rolling element bearings: A review, Advances in Mechanical Engineering, (2018).
[5] J. Liu and Y. Shao, Overview of dynamic modeling and analysis of rolling element bearings with localized and distributed faults, Nonlinear Dynamics, (2018).
[6] H. Cao, L. Niu, S. Xi, and X. Chen, Mechanical model development of rolling bearing-rotor systems: A review, Mechanical Systems, and Signal Processing, 102 (2018) 37–58.
[7] I. Howard, A Review of Rolling Element Bearing Vibration ‘Detection, Diagnosis, and Prognosis, Defence Science and Technology Organization, Canberra, Australia, DSTO-RR-0013, (1994).
[8] N. Tandon and A. Choudhury, A review of vibration and acoustic measurement methods for the detection of defects in rolling element bearings, Tribology International, 32(8) (1999) 469–480.
[9] M. S. Patil, J. Mathew, and P. K. Rajendra Kumar, Bearing Signature Analysis as a Medium for Fault Detection: A Review, Journal of Tribology, 130(1) (2008).
[10] H. S. Kumar, P. P. Shrinivasa, G. S. Vijay, B. K. N. Rao, Wavelet transform for bearing condition monitoring and fault diagnosis: A review, International Journal of COMADEM, 17 (2013).
[11] S. Patidar and P. K. Soni, An Overview on Vibration Analysis Techniques for the Diagnosis of Rolling Element Bearing Faults, International Journal of Engineering Trends and Technology, (2013).
[12] T. Lin, K. Yu, and J. Tan, Condition Monitoring and Fault Diagnosis of Roller Element Bearing, in Bearing Technology, (2017).
[13] P. Gupta and M. K. Pradhan, Fault detection analysis in rolling element bearing: A review, Materials Today: Proceedings, 4(2) (2017) 2085–2094.
[14] C. Malla and I. Panigrahi, Review of Condition Monitoring of Rolling Element Bearing Using Vibration Analysis and Other Techniques, Journal of Vibration Engineering and Technology, 7(4) (2019) 407–414.
[15] H. Patidar and R. K. Mandloi, Study of Detection of Defects in Rolling Element Bearings using Vibration and Acoustic Measurement Methods - A Review, International Research Journal of Engineering and Technology, 5(3)(2015) 17.
[16] Mohd Syahril Ramadhan Saufi, Z. A. Ahmad, M. H. Lim, and M. S. Leong, A review on signal processing techniques for bearing diagnostics, International Journal of Mechanical Engineering and Technology, 8(6)(2017) 327–337.
[17] A. Rai and S. H. Upadhyay, A review on signal processing techniques utilized in the fault diagnosis of rolling element bearings, Tribology International, 96(2016) 289–306.
[18] P. H. Jain and S. P. Bhosle, Analysis on VSA Techniques used in Diagnosis of Faults in Rotating Machinery, International Journal of Mechanical and Production Engineering Research and Development, 10(3)(2020) 3370–3396.
[19] M. Amarnath, R. Shrinidhi, A. Ramachandra, and S. B. Kandagal, Prediction of Defects in Antifriction Bearings using Vibration Signal Analysis, Journal of the Institution of Engineers (India), (2004).
[20] T. Karacay and N. Akturk, Experimental diagnostics of ball bearings using statistical and spectral methods, Tribology International, 42(6) (2009) 836–843.
[21] P. Jayaswal and S. N. Verma, Application of vibration signature analysis techniques for rolling element bearing fault identification, Australian Journal of Mechanical Engineering, 8(1)(2011) 21–36.
[22] D. Dyer and R. M. Stewart, Detection of Rolling Element Bearing Damage by Statistical Vibration Analysis, Journal of Mechanical Design, 100(2)(1978) 229–235.
[23] N. Tandon, A comparison of some vibration parameters for the condition monitoring of rolling element bearings, Measurement, 12(3)(1994) 285–289.
[24] H. R. Martin and F. Honarvar, Application of statistical moments to bearing failure detection, Applied Acoustics, 44(1)(1995) 67–77.
[25] R. B. W. Heng and M. J. M. Nor, Statistical analysis of sound and vibration signals for monitoring rolling element bearing condition, Applied Acoustics, 53(1) (1998) 211–226.
[26] R. G. T. de Almeida, S. A. da Silva Vicente, and L. R. Padovese, New Technique for Evaluation of Global Vibration Levels in Rolling Bearings, Shock and Vibration, (2002).
[27] A. Utpat, R. B. Ingle, and M. R. Nandgaonkar, Response of Various Vibration Parameters to the Condition Monitoring of Ball Bearing Used in Centrifugal Pumps, Noise & Vibration Worldwide, 42(6) (2011) 34–40.
[28] X. Niu, L. Zhu, and H. Ding, New statistical moments for the detection of defects in rolling element bearings, International Journal of Advanced Manufacturing Technology, 26(11) (2005) 1268–1274.
[29] B. Tao, L. Zhu, H. Ding, and Y. Xiong, An alternative time-domain index for condition monitoring of rolling element bearings – A comparison study, Reliability Engineering & System Safety, 92(5) (2007) 660–670.
[30] S. Sassi, B. Badri, and M. Thomas, Tracking surface degradation of ball bearings by means of new time-domain scalar indicators, International Journal of COMADEM, 11(3)(2008) 36–45.
[31] D. Paliwal, A. Choudhury, and G. Tingarikar, Wavelet and Scalar Indicator based Fault Assessment Approach for Rolling Element Bearings, Procedia Materials Science, 5(2014).
[32] T. I. Liu and J. M. Mengel, Intelligent monitoring of ball bearing conditions, Mechanical Systems and Signal Processing, 6(5)(1992) 419–431.
[33] B. Samanta and K. R. Al-balushi, ANN-based fault diagnostics of rolling element bearings using time-domain features, Mechanical Systems and Signal Processing, 17(2)(2003) 317–328.
[34] B. Sreejith, A. K. Verma, and A. Srividya, Fault diagnosis of rolling element bearing using time-domain features and neural networks, in 2008 IEEE Region 10 and the Third International Conference on Industrial and Information Systems, (2008) 1–6.
[35] V. Hariharan and P. Srinivasan, New Approach of Classification of Rolling Element Bearing Fault using Artificial Neural Network, Journal of Mechanical Engineering, 40(2) (2009).
[36] J. P. Patel and S. H. Upadhyay, Comparison between Artificial Neural Network and Support Vector Method for a Fault Diagnostics in Rolling Element Bearings, Procedia Engineering, 144 (2016) 390–397.
[37] B. S. Kim, S. H. Lee, M. G. Lee, J. Ni, J. Y. Song, and C. W. Lee, A comparative study on damage detection in speed-up and coast-down process of grinding spindle-typed rotor-bearing system, Journal of Materials Processing Technology, 187–188(2007) 30–36.
[38] T. A. Harris, Rolling Bearing Analysis, Third Edition. John Wily and Son, INC., (1991).
[39] D. S. Shah, V. N. Patel, and P. H. Darji, Experimental Vibration Studies of Deep Groove Ball Bearings Having Damaged Surfaces, Journal of The Institution of Engineers (India): Series C, 100(6) (2019) 919–935.
[40] J. I. Taylor, Identification of Bearing Defects by Spectral Analysis, Journal of Mechanical Design, 102(2)(1980) 199–204.
[41] T. Igarashi and S. Yabe, Studies on the Vibration and Sound of Defective Rolling Bearings : Second Report : Sound of Ball Bearings with One Defect, Bulletin of JSME, 26(220)(1983) 1791–1798.
[42] N. Tandon and K. S. Kumar, Detection of Defects at Different Locations in Ball Bearings by Vibration and Shock Pulse Monitoring, Noise & Vibration Worldwide, (2003).
[43] J. Liu, Y. Shao, and M. J. Zuo, The effects of the shape of the localized defect in ball bearings on the vibration waveform: Proceedings of the Institution of Mechanical Engineers, Part K: Journal of Multi-body Dynamics, (2013).
[44] S. Orhan, N. Aktürk, and V. Çelik, Vibration monitoring for defect diagnosis of rolling element bearings as a predictive maintenance tool: Comprehensive case studies, NDT & E International, 39(4) (2006) 293–298.
[45] V. N. Patel, N. Tandon, and R. K. Pandey, Vibration Studies of Dynamically Loaded Deep Groove Ball Bearings in the presence of Local Defects on Races, Procedia Engineering, 64 (2013) 182–1591.
[46] V. N. Patel, N. Tandon, and R. K. Pandey, Experimental Study for Vibration Behaviors of Locally Defective Deep Groove Ball Bearings under Dynamic Radial Load, Advances in Acoustics and Vibration, (2014).
[47] V. N. Patel, N. Tandon, and R. K. Pandey, Vibrations Generated by Rolling Element Bearings having Multiple Local Defects on Races, Procedia Technology, 14(2014) 312–319.
[48] A. Khadersab and S. Shivakumar, Vibration Analysis Techniques for Rotating Machinery and its effect on Bearing Faults, Procedia Manufacturing, 20(2018) 247–252.
[49] J. Semmlow, The Fourier Transform, and Power Spectrum, in Signals and Systems for Bioengineers, (2012) 131–165.
[50] M. Feldman, Hilbert transform applications in mechanical vibration. John Wiley & Sons Ltd.,(2011).
[51] R. B. Randall and J. Antoni, Rolling element bearing diagnostics—A tutorial, Mechanical Systems, and Signal Processing, 25(2)(2011) 485–520.
[52] G. Feng, J. Gu, D. Zhen, M. Aliwan, F. Gu, and A. Ball, Implementation of envelope analysis on a wireless condition monitoring system for bearing fault diagnosis, International Journal of Automation and Computing, 12(2015) 14–24.
[53] B. Betea, P. Dobra, M. C. Gherman, and L. Tomesc, Comparison between envelope detection methods for bearing defects diagnosis, IFAC Proceedings Volumes, 46(6)(2013) 137–142.
[54] S. Alegranzi, J. Fagundes Gonçalves, and H. Gomes, Ball bearing vibration monitoring for fault detection by the envelope technique, Sao Paulo Brazil, (2014) 4113–4125.
[55] B. S. Kim, D. S. Gu, J. G. Kim, Y. C. Kim, and B. K. Choi, Rolling element bearing fault detection using acoustic emission signal analyzed by envelope analysis with discrete wavelet transform, in Engineering Asset Lifecycle Management, London, (2010) 596–602.
[56] N. Wang and X. Liu, Bearing Fault Diagnosis Method Based on Hilbert Envelope Demodulation Analysis, IOP Conference Series: Material Science and Engineering, 436(2018) 012009.
[57] Y. Amirat, V. V. Choqueuse, M. Benbouzid, and S. Turri, Hilbert Transform-Based Bearing Failure Detection in DFIG-Based Wind Turbines, International Review of Electrical Engineering,6(3)(2011) 1249–1256.
[58] G. A. Jiménez, A. O. Muñoz, and M. A. Duarte-Mermoud, Fault detection in induction motors using Hilbert and Wavelet transform, Electrical Engineering , 89(3)(2007) 205–220.
[59] V. N. Patel, N. Tandon, and R. Pandey Defect detection in deep groove ball bearing in the presence of external vibration using envelope analysis and Duffing oscillator, Measurement, 45(2012) 960–970.
[60] Z. Yang, U. C. Merrild, M. T. Runge, G. Pedersen, and H. Børsting, A Study of Rolling-Element Bearing Fault Diagnosis Using Motor’s Vibration and Current Signatures, IFAC Proceedings Volumes, 42(8)(2009) 354–359.
[61] C. S. Park, Y. C. Choi, and Y.-H. Kim, Early fault detection in automotive ball bearings using the minimum variance cepstrum, Mechanical Systems, and Signal Processing, 38(2)(2013) 534–548.
[62] Y. R. Hwang, K. K. Jen, and Y.-T. Shen, Application of cepstrum and neural network to bearing fault detection, Journal of Mechanical Science and Technology, 23(10) (2009) 2730–2737.
[63] M. E. Morsy and G. Achtenová, Rolling bearing fault diagnosis techniques - autocorrelation and cepstrum analyses, in 2015 23rd Mediterranean Conference on Control and Automation (MED), (2015) 328–334.
[64] C. Peeters, P. Guillaume, and J. Helsen, Signal pre-processing using cepstral editing for vibration-based bearing fault detection, presented at the International Conference on Noise and Vibration Engineering, Belgium,(2016).
[65] N. Sawalhi, W. Wang, and A. Becker, Vibration Signal Processing Using Cepstrum Editing Technique to Enhance Spall-related Vibration Features in Rolling Element Bearings, International Journal of Mechanical Engineering and Robotics Research,(2018) 65–68.
[66] I. Bediaga, X. Mendizabal, A. Arnaiz, and J. Munoa, Ball bearing damage detection using traditional signal processing algorithms, IEEE Instrumentation Measurement Magazine, 16(2)(2013) 20–25.
[67] E. Elbouchikhi, V. Choqueuse, M. Benbouzid, J. F. Charpentier, and G. Barakat, A Comparative Study of Time-Frequency Representations for Fault Detection in Wind Turbine,(2012).
[68] F. Al-Badour, M. Sunar, and L. Cheded, Vibration analysis of rotating machinery using time-frequency analysis and wavelet techniques, Mechanical Systems and Signal Processing, 25(6)(2011) 2083–2101.
[69] F. Fan and M. Zuo, Machine fault feature extraction based on intrinsic mode functions, Measurement Science and Technology, 19 (2008) 045105(12p).
[70] Z. Feng, M. Liang, and F. Chu, Recent advances in time-frequency analysis methods for machinery fault diagnosis: A review with application examples, Mechanical Systems and Signal Processing, 38(1)(2013) 165–205.
[71] H. Li, Q. He, and Z. Zhang, Overview of Time-Frequency Analysis Techniques in Vibration Signals of Rotating Machinery, Applied Mechanics and Materials, 684(2014) 124–130.
[72] S. Li, Y. Xin, X. Li, J. Wang, and K. Xu, A Review on the Signal Processing Methods of Rotating Machinery Fault Diagnosis, in 2019 IEEE 8th Joint International Information Technology and Artificial Intelligence Conference (ITAIC),(2019) 1559–1565.
[73] K. M. Hui, L. M. Hee, and M. S. Leong, Time-Frequency Signal Analysis in Machinery Fault Diagnosis : Review, Advanced Materials Research, 845(2014) 41–45.
[74] A. Lakis, Rotating Machinery Fault Diagnosis using Time-Frequency Methods, 7th WSEAS International Conference on Electric Power Systems, High Voltages, Electric Machines, Venice, Italy, (2007) 139–144.
[75] R. B. Randall, Vibration-based Condition Monitoring. A John Wiley and Sons Ltd Publication, (2011).
[76] A. H. Boudinar, A. F. Aimer, M. E. A. Khodja, and N. Benouzza, Induction Motor’s Bearing Fault Diagnosis Using an Improved Short Time Fourier Transform, in Advanced Control Engineering Methods in Electrical Engineering-Systems, Cham, (2019) 411–426.
[77] M. Cocconcelli, R. Zimroz, R. Rubini, and W. Bartelmus, STFT Based Approach for Ball Bearing Fault Detection in a Varying Speed Motor, in Condition Monitoring of Machinery in Non-Stationary Operations, Berlin, Heidelberg, (2012) 41–50.
[78] H. Gao, L. Liang, X. Chen, and G. Xu, Feature extraction and recognition for rolling element bearing fault utilizing short-time Fourier transform and non-negative matrix factorization, Chinese Journal of Mechanical Engineering, 28(2015) 96–105.
[79] H. Liu, L. Li, and J. Ma, Rolling Bearing Fault Diagnosis Based on STFT-Deep Learning and Sound Signals, Shock and Vibration, 2016 (2016).
[80] H. Bendjama, S. Bouhouche, and M. S. Boucherit, Application of Wavelet Transform for Fault Diagnosis in Rotating Machinery,
International Journal of Machine Learning and Computing IJMLC, 2(1)(2012) 82–87.
[81] A. Boudiaf, Z. Mentouri, S. Ziani, S. Taleb, and A. Moussaoui, Wavelet Transform And Envelope Detection For Gear Fault Diagnosis. A Comparative Study, (2013).
[82] G. G. Yen and K.-C. Lin, Wavelet packet feature extraction for vibration monitoring, IEEE Transactions on Industrial Electronics, 47(3) (2000) 650–667.
[83] S. Kulkarni, A. Bewoor, and R. Ingle, Vibration signature analysis of distributed defects in ball bearing using wavelet decomposition technique, Noise & Vibration Worldwide, 48(1–2)(2017) 7–18.
[84] P. K. Kankar, S. C. Sharma, and S. P. Harsha, Rolling element bearing fault diagnosis using wavelet transform, Neurocomputing, 74(10)(2011) 1638–1645.
[85] Y. Yunlong and Z. Zhenxiang, Fault diagnosis of rolling bearing based on the wavelet analysis, in Proceedings of the 2nd international Asia conference on Informatics in control, automation and robotics - Vol. - 1, Wuhan, China, (2010) 257–260.
[86] S. Prabhakar, A. R. Mohanty, and A. S. Sekhar, Application of discrete wavelet transform for detection of ball bearing race faults, Tribology International, 35(2002) 793–800,
[87] K. Mori, N. Kawashima, T. Yoshioka, and Y. Ueno, Prediction of spalling on a ball bearing by applying the discrete wavelet transform to vibration signals, Wear, 195(1)(1996) 162–168.
[88] R. Kumar and M. Singh, Outer race defect width measurement in taper roller bearing using discrete wavelet transform of the vibration signal, Measurement, 46(1)(2013) 537–545.
[89] S. Khanam, N. Tandon, and J. K. Dutt Fault Size Estimation in the Outer Race of Ball Bearing Using Discrete Wavelet Transform of the Vibration Signal, Procedia Technology, 14 (2014) 12–19.
[90] J. Chebil, M. Hrairi, and N. M. Abu-Shikhah, Signal Analysis of Vibration Measurements for Condition Monitoring of Bearings, Australian Journal of Basic and Applied Sciences, 5(1)(2011) 70–78.
[91] N. G. Nikolaou and I. A. Antoniadis, Rolling element bearing fault diagnosis using wavelet packets, NDT & E International, 35(3) (2002) 197–205.
[92] P. G. Kulkarni and A. D. Sahasrabudhe, Application Of Wavelet Transform For Fault Diagnosis of Rolling Element Bearings, International Journal of Scientific & Technology, 2(4)(2013) 138–148.
[93] D. H. Pandya, S. H. Upadhyay, and S. P. Harsha, ANN Based Fault Diagnosis of Rolling Element Bearing Using Time-Frequency Domain Feature, International Journal of Engineering Science and Technology, 4(6)(2012) 2878–2886.
[94] C. K. E. Nizwan, S. A. Ong, M. F. M. Yusof, and M. Z. Baharom, A wavelet decomposition analysis of vibration signal for bearing fault detection, IOP Conf. Ser.: Mater. Sci. Eng., 50(2013) 012026(9p).
[95] P. Singru, V. Krishnakumar, D. Natarajan, and A. Raizada, Bearing failure prediction using Wigner-Ville distribution, modified Poincare mapping and fast Fourier transform, Journal of Vibroengineering, 20 (2018).
[96] Q. Meng and L. Qu, Rotating machinery fault diagnosis using Wigner distribution, Mechanical Systems and Signal Processing, 5(3)(1991) 155–166.
[97] H. Li, H. Zheng, and L. Tang, Wigner-Ville distribution based on EMD for faults diagnosis of bearing, in Proceedings of the Third international conference on Fuzzy Systems and Knowledge Discovery, China, (2006) 803–812.
[98] Y. Zhou, J. Chen, G. M. Dong, W. B. Xiao, and Z. Y. Wang, Wigner–Ville distribution based on cyclic spectral density and the application in rolling element bearings diagnosis: Proceedings of the Institution of Mechanical Engineers, Part C: Journal of Mechanical Engineering Science, (2011).
[99] H. Wang and Y. Ji, A Revised Hilbert–Huang Transform and Its Application to Fault Diagnosis in a Rotor System, Sensors, 18(12) (2018) 4329(27).
[100] N. E. Huang et al., The empirical mode decomposition and the Hilbert spectrum for nonlinear and non-stationary time series analysis, Proceedings of the Royal Society of London. Series A: Mathematical, Physical and Engineering Sciences, 454(1971) (1998) 903–995.
[101] P. S. Kumar, L. A. Kumaraswamidhas, and S. K. Laha Selecting effective intrinsic mode functions of empirical mode decomposition and variational mode decomposition using dynamic time warping algorithm for rolling element bearing fault diagnosis, Transactions of the Institute of Measurement and Control, 41(7)(2019) 1923–1932.
[102] D. Yu, J. Cheng, and Y. Yu, Application of EMD method and Hilbert spectrum to the fault diagnosis of roller bearings, Mechanical Systems and Signal Processing, 19 (2005) 259–270.
[103] V. K. Rai and A. R. Mohanty, Bearing fault diagnosis using FFT of intrinsic mode functions in Hilbert–Huang transform, Mechanical Systems & Signal Processing, 21(6)(2007) 2607-2615.
[104] H. Li, Y. Zhang, and H. Zheng, Hilbert-Huang transform and marginal spectrum for detection and diagnosis of localized defects in roller bearings, Journal of Mechanical Science and Technology, 23(2)(2009) 291–301.
[105] A. Kabla and K. Mokrani, Bearing fault diagnosis using Hilbert-Huang transform (HHT) and support vector machine (SVM), Mechanics & Industry, 17(3)(2016) 308 (10p).
[106] T. N. Babu, S. Devendiran, A. Aravind, A. Rakesh, and M. Jahzan, Fault Diagnosis on Journal Bearing Using Empirical Mode Decomposition, Materials Today: Proceedings, 5(5)(2018) 12993–13002.
[107] R. Yan and R. X. Gao, Hilbert–Huang Transform-Based Vibration Signal Analysis for Machine Health Monitoring, IEEE Transactions on Instrumentation and Measurement, 55(6)(2006) 2320–2329.
[108] F. Chen, X. Zhou, Q. Wu, T. He, and H. He, Application of Hilbert-Huang transformation to fault diagnosis of rotary machinery, presented at the Fifth International Symposium on Instrumentation Science and Technology,(2009).
[109] E. Elbouchikhi, V. Choqueuse, Y. Amirat, M. Benbouzid, and S. Turri, An Efficient Hilbert-Huang Transform-based Bearing Faults Detection in Induction Machines, IEEE Transactions on Energy Conversion,(2017).
[110] Z. K. Peng, P. W. Tse, and F. L. Chu, A comparison study of improved Hilbert–Huang transform and a wavelet transform Application to fault diagnosis for rolling bearing, Mechanical Systems & Signal Processing, 19(5)(2005) 974-988.
[111] Z. K. Peng, P. W. Tse, and F. L. Chu, An improved Hilbert–Huang transform and its application in vibration signal analysis, Journal of Sound and Vibration, 286(1)(2005) 187–205.
[112] S. Osman and W. Wang, An enhanced Hilbert–Huang transform technique for bearing condition monitoring, Measurement Science and Technology, 24,(2013) 085004.
[113] S. Osman and W. Wang, A normalized Hilbert-Huang transform technique for bearing fault detection: Journal of Vibration and Control, (2014).
[114] Ping Huang, Ziwei Pan, Xiaoli Qi, and Jiapeng Lei, Bearing fault diagnosis based on EMD and PSD, in 2010 8th World Congress on Intelligent Control and Automation, (2010) 1300–1304.
[115] Y. Lei, J. Lin, Z. He, and M. J. Zuo, A review on empirical mode decomposition in fault diagnosis of rotating machinery, Mechanical Systems and Signal Processing, 35(1)(2013) 108–126.
[116] M. F. Isham, M. Leong, L. M. Hee, and Z. Ahmad, Empirical mode decomposition: A review on model selection method for rotating machinery diagnosis, International Journal of Mechanical Engineering and Technology, 8(2017) 16–26.
[117] Y. Yang, Y. Dejie, and C. Junsheng, A fault diagnosis approach for roller bearing based on IMF envelope spectrum and SVM, Measurement, 40(2007) 943–950.
[118] C. Junsheng, Y. Dejie, and Y. Yu, The application of energy operator demodulation approach based on EMD in machinery fault diagnosis, Mechanical Systems, and Signal Processing, 21(2)(2007) 668–677.
[119] R. Yan and R. X. Gao, Rotary Machine Health Diagnosis Based on Empirical Mode Decomposition, Journal of Vibration and Acoustics, 130(2)(2008) 021007(12p).
[120] D. Wei and L. Quan, A New Ball Bearing Fault Diagnosis Method Based on EMD and SVM, in Advanced Electrical and Electronics Engineering, Berlin, Heidelberg, (2011) 423–428.
[121] P. Singh and S. P. Harsha, Statistical and frequency analysis of vibrations signals of roller bearings using empirical mode decomposition: Proceedings of the Institution of Mechanical Engineers, Part K: Journal of Multi-body Dynamics, (2019).
[122] Q. Du and S. Yang, Application of the EMD method in the vibration analysis of ball bearings, Mechanical Systems and Signal Processing, 21(6)(2007) 2634–2644.
[123] H. Dong, K. Qi, X. Chen, Y. Zi, Z. He, and B. Li, Sifting process of EMD and its application in rolling element bearing fault diagnosis, Journal of Mechanical Science and Technology, 23(8)(2009) 2000–2007.
[124] T. Han, D. Jiang, and N. Wang, The Fault Feature Extraction of Rolling Bearing Based on EMD and Difference Spectrum of Singular Value, Shock and Vibration, (2016) 1–14.
[125] C. Delprete, E. Brusa, C. Rosso, and F. Bruzzone, Bearing Health Monitoring Based on the Orthogonal Empirical Mode Decomposition, Shock and Vibration, (2020) 1–9.
[126] Z. Wu and N. E. Huang, Ensemble empirical mode decomposition: a noise-assisted data analysis method, Adv. Adapt. Data Anal., 1(1) (2009) 1–41.
[127] H. Li, Y. Wang, and Y. Ma, Ensemble empirical mode decomposition and Hilbert-Huang transform applied to bearing fault diagnosis, in 2010 3rd International Congress on Image and Signal Processing, 7(2010) 3413–3417.
[128] T.Y. Wu, H.C. Hong, and Y.L. Chung, A looseness identification approach for rotating machinery based on post-processing of ensemble empirical mode decomposition and autoregressive modeling: Journal of Vibration and Control, (2011).
[129] J. M. Lu, F. L. Meng, H. Shen, L. B. Ding, and S. N. Bao, Fault Diagnosis of Rolling Bearing Based on EEMD and Instantaneous Energy Density Spectrum, Applied Mechanics and Materials, 142 (2011) 741–744.
[130] Y. Chang, F. Jiang, Z. Zhu, and W. Li, Fault diagnosis of rotating machinery based on time-frequency decomposition and envelope spectrum analysis, Journal of Vibroengineering, 19(2017) 943–954.
[131] X. Qin, Q. Li, X. Dong, and S. Lv, The Fault Diagnosis of Rolling Bearing Based on Ensemble Empirical Mode Decomposition and Random Forest, Shock, and Vibration, (2017) 1–9.
[132] C. Gao, T. Wu, and Z. Fu, Advanced Rolling Bearing Fault Diagnosis Using Ensemble Empirical Mode Decomposition, Principal Component Analysis and Probabilistic Neural Network, Journal of Robotics, Networking, and Artificial Life, 5(1)(2018) 10–14.
[133] Z. Feng, M. J. Zuo, R. Hao, F. Chu, and J. Lee, Ensemble Empirical Mode Decomposition-Based Teager Energy Spectrum for Bearing Fault Diagnosis, Journal of Vibration and Acoustics 135(3)(2013).
[134] V. Verma, J. S. Ratol, and A. Singh, Bearing Fault Detection using Ensemble Empirical Mode Decomposition integrated with Teager Kaiser Energy Method, International Journal of Technology and Computing, 4(12)(2018) 167–171.
[135] J. Xiang and Y. Zhong, A new fault detection strategy using the enhancement ensemble empirical mode decomposition, Journal of Physics: Conference Series, 842 (2017) 012002(10p).
[136] Y. Cheng, Z. Wang, B. Chen, W. Zhang, and G. Huang, An improved complementary ensemble empirical mode decomposition with adaptive noise and its application to rolling element bearing fault diagnosis, ISA Transactions, 91(2019) 218–234.
[137] D. Zhen, J. Guo, Y. Xu, H. Zhang, and F. Gu, A Novel Fault Detection Method for Rolling Bearings Based on Non-Stationary VSA, Sensors, 19(18)(2019) Art. no. 18.
[138] J. S. Smith, The local mean decomposition and its application to EEG perception data, J R Soc Interface, 2(5) (2005) 443–454.
[139] B. Chen, X. Chen, Z. He, and J. Tan, Mechanical Fault Diagnosis Based on Local Mean Decomposition Method, Measuring Technology and Mechatronics Automation, International Conference on, 1 (2009) 681–684.
[140] H. Li, Fault Diagnosis of Gear Wear Based on Local Mean Decomposition, Advanced Materials Research, 459 (2012) 298–302.
[141] Y. Wang, Z. He, and Y. Zi, A Comparative Study on the Local Mean Decomposition and Empirical Mode Decomposition and Their Applications to Rotating Machinery Health Diagnosis, Journal of Vibration and Acoustics, 132(2) (2010) 021010(10p).
[142] L. Yongbo, S. Shubin, L. Zhiliang, and L. Xihui, Review of local mean decomposition and its application in fault diagnosis of rotating machinery, Journal of Systems Engineering and Electronics, 30(4) (2019) 799–814.
[143] B. Chen, Z. He, X. Chen, H. Cao, G. Cai, and Y. Zi, A demodulating approach based on local mean decomposition and its applications in mechanical fault diagnosis, Measurement Science and Technology, 22(5)(2011) 055704.
[144] H. Li, Local Mean Decomposition Based Bearing Fault Detection, Advanced Materials Research, 490–495 (2012) 360–364.
[145] J. Cheng, Y. Yang, and Y. Yang, A rotating machinery fault diagnosis method based on local mean decomposition, Digital Signal Processing, 22(2)(2012) 356–366.
[146] J. Ma, J. Wu, and X. Yuan, The Fault Diagnosis of the Rolling Bearing Based on the LMD and Time-frequency Analysis, International Journal of Control and Automation, 6(4)(2013) 357–376.
[147] W. Jiying and L. Zhenxing, A Self-adaptive Analysis Method of Fault Diagnosis in Roller Bearing Based on Local Mean Decomposition, in The 26th Chinese Control and Decision Conference (2014) 218–222.
[148] Y. Li, M. Xu, Z. Haiyang, Y. Wei, and W. Huang, A New Rotating Machinery Fault Diagnosis Method Based on Improved LMD, Digital Signal Processing, 46(2015) 201–214.
[149] J. Ma, J. Wu, Y. Fan, and X. Wang, The Rolling Bearing Fault Feature Extraction Based on the LMD and Envelope Demodulation, Mathematical Problems in Engineering,(2015) 1–13.
[150] L. Yanqiang and J. Jie, Fault diagnosis of bearing based on LMD and MSE, in 2017 Prognostics and System Health Management Conference (PHM-Harbin), (2017) 1–4.
[151] Z. Liu, Y. Jin, M. J. Zuo, and Z. Feng, Time-frequency representation based on robust local mean decomposition for multi-component AM-FM signal analysis, Mechanical Systems and Signal Processing, 95(2017) 468–487.
[152] L. Wang, Z. Liu, Q. Miao, and X. Zhang, Complete ensemble local mean decomposition with adaptive noise and its application to fault diagnosis for rolling bearings, Mechanical Systems and Signal Processing, 106(2018) 24–39.
[153] L. Wang, Z. Liu, Q. Miao, and X. Zhang, Time-frequency analysis based on ensemble local mean decomposition and fast kurtogram for rotating machinery fault diagnosis, Mechanical Systems, and Signal Processing, 103(2018) 60–75.
[154] C. Yao, D. Zou, W. Zhang, and Z. Wang, A Hybrid Time-Frequency Analysis Method for Railway Rolling-Element Bearing Fault Diagnosis, Journal of Sensors, (2019) 1–12.
[155] P. Rao and N. Saralika, Thermal and vibration analysis of 6200 deep groove ball bearing, International Journal of Mechanical Engineering, (2017) 76–81.