Abstract
A Machine learning-based approach for developing an app capable of recognizing and disseminating healthcare data. Among the world, the major cause of disability is stroke. Brain ischemia subgroup was crucial not only for effective mediation and care, but also for the visualization of injury. An integrated form was used to organize the subcategories of brain ischemia on the global clot trail data in this study. Initially, the Shapiro-Wilk calculation was used to determine the importance of highlights, as well as Pearson relationships between highlights. Early finding of stroke is fundamental for opportune counteraction and treatment. Information was gathered from International Stroke Trial data set and was effectively prepared and tried utilizing Sequential Minimal Optimization. At that point, we utilized Recursive Feature Elimination with Cross-Validation, which conglomerate direct SVC, Random decision Forest Classifier, Extremely-Randomized Trees Classifier, Adobos-Classifier, and Multivariate Event model Classifier as assessor individually, to choose hearty highlights imperative to brain ischemia subgrouping. Moreover, the significance of chose highlights was controlled by Extra Trees-Classifier. At long last, the chose highlights were utilized by Extra-Trees-Classifier.
Export citation and abstract BibTeX RIS
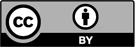
Content from this work may be used under the terms of the Creative Commons Attribution 3.0 licence. Any further distribution of this work must maintain attribution to the author(s) and the title of the work, journal citation and DOI.
This article (and all articles in the proceedings volume relating to the same conference) has been retracted by IOP Publishing following an extensive investigation in line with the COPE guidelines. This investigation has uncovered evidence of systematic manipulation of the publication process and considerable citation manipulation.
IOP Publishing respectfully requests that readers consider all work within this volume potentially unreliable, as the volume has not been through a credible peer review process.
IOP Publishing regrets that our usual quality checks did not identify these issues before publication, and have since put additional measures in place to try to prevent these issues from reoccurring. IOP Publishing wishes to credit anonymous whistleblowers and the Problematic Paper Screener [1] for bringing some of the above issues to our attention, prompting us to investigate further.
[1] Cabanac G, Labbé C and Magazinov A 2021 arXiv:2107.06751v1
Retraction published: 23 February 2022