Abstract
Time delay neural networks (TDNNs) have been shown to be an efficient network architecture for modelling long temporal contexts in speech recognition. Meanwhile, the training times of TDNNs are much less, compared with other long temporal contexts models based on recurrent neural networks. In this paper, we propose deeper architectures to improve the modelling power of TDNNs. At each TDNN layer that needs spliced input, we increase the number of transforms so that the lower layers can provide more salient features for upper layers. Dropout is found to be an effective way to prevent the model from overfitting once the depth of the model is substantially increased. The proposed architectures significantly improvements the recognition accuracy in Switchboard and AMI.
Export citation and abstract BibTeX RIS
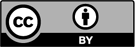
Content from this work may be used under the terms of the Creative Commons Attribution 3.0 licence. Any further distribution of this work must maintain attribution to the author(s) and the title of the work, journal citation and DOI.