Abstract
Purposes
Many factors were reported to be associated with diabetic retinopathy (DR); however, their contributions remained unclear. We aimed to evaluate the prognostic and diagnostic accuracy of logistic regression and three machine learning models based on various medical records.
Methods
This was a cross-sectional study. We investigated the prevalence and associations of DR among 757 participants aged 40 years or older in the 2005–2006 National Health and Nutrition Examination Survey (NHANES). We trained the models to predict if the participants had DR with 15 predictor variables. Area under the receiver operating characteristic (AUROC) and mean squared error (MSE) of each algorithm were compared in the external validation dataset using a replicate cohort from NHANES 2007–2008.
Results
Among the 757 participants, 53 (7.00%) subjects had DR, the mean (standard deviation, SD) age was 57.7 (13.04), and 78.0% were male (n = 42). Logistic regression revealed that female gender (OR = 4.130, 95% CI: 1.820–9.380; P < 0.05), HbA1c (OR = 1.665, 95% CI: 1.197–2.317; P < 0.05), serum creatine level (OR = 2.952, 95% CI: 1.274–6.851; P < 0.05), and eGFR level (OR = 1.009, 95% CI: 1.000–1.014, P < 0.05) increased the risk of DR. The average performance obtained from internal validation was similar in all models (AUROC ≥ 0.945), and k-nearest neighbors (KNN) had the highest value with an AUROC of 0.984. In external validation, they remained robust or with modest reductions in discrimination with AUROC still ≥ 0.902, and KNN also performed the best with an AUROC of 0.982. Both logistic regression and machine learning models had good performance in the clinical diagnosis of DR.
Conclusions
This study highlights the utility of comparing traditional logistic regression to machine learning models. We found that logistic regression performed as well as optimized machine learning methods when classifying DR patients.




Similar content being viewed by others
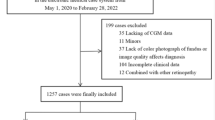
Data availability
Data were acquired from the National Health and Nutrition Examination Survey (https://www.cdc.gov/nchs/nhanes/).
Abbreviations
- DR:
-
Diabetic retinopathy
- Scr:
-
Serum creatine
- eGFR:
-
Estimated glomerular filtration rate
- NHANES:
-
National Health and Nutrition Examination Survey
- AUROC:
-
Area under the receiver operating characteristic
- MSE:
-
Mean squared error
- SD:
-
Standard deviation
- OR:
-
Odds ratio
- KNN:
-
K-nearest neighbors
- NPDR:
-
Non-proliferative diabetic retinopathy
- PDR:
-
Proliferative diabetic retinopathy
- LR:
-
Logistic regression
- RF:
-
Random forest
- SVM:
-
Support vector machine
References
Yau JWY, Rogers SL, Kawasaki R et al (2012) Global prevalence and major risk factors of diabetic retinopathy. Diabetes Care 35:556–564
Cheung N, Mitchell P, Wong TY (2010) Diabetic retinopathy. Lancet 376:124–136
Hainsworth DP, Bebu I, Aiello LP et al (2019) Risk factors for retinopathy in type 1 diabetes. Diabetes Care 42:875–882
Ting DSW, Cheung GCM, Wong TY (2016) Diabetic retinopathy: global prevalence, major risk factors, screening practices and public health challenges: a review. Clin Exp Ophthalmol 44:260–277
Ting DSW, Cheung CYL, Lim G et al (2017) Development and validation of a deep learning system for diabetic retinopathy and related eye diseases using retinal images from multiethnic populations with diabetes. JAMA 318:2211–2223
Lo-Ciganic WH, Huang JL, Zhang HH et al (2019) Evaluation of machine-learning algorithms for predicting opioid overdose risk among medicare beneficiaries with opioid prescriptions. JAMA Netw open 2:e190968
Wong A, Young AT, Liang AS et al (2018) Development and validation of an electronic health record-based machine learning model to estimate delirium risk in newly hospitalized patients without known cognitive impairment. JAMA Netw open 1:e181018
Frizzell JD, Liang L, Schulte PJ et al (2017) Prediction of 30-day all-cause readmissions in patients hospitalized for heart failure: comparison of machine learning and other statistical approaches. JAMA Cardiol 2:204–209
Wong WL, Su X, Li X et al (2014) Global prevalence of age-related macular degeneration and disease burden projection for 2020 and 2040: a systematic review and meta-analysis. Lancet Glob Heal 2:e106–e116
World Medical Association (2013) Ethical principles for medical research involving human subjects. JAMA 310:2191–2194
Flaxel CJ, Adelman RA, Bailey ST et al (2020) Diabetic retinopathy preferred practice pattern. Ophthalmol 127:66–145
Meurer WJ, Juliana T (1986) Logistic regression diagnostics understanding how well a model predicts outcomes. J Am Stat Assoc 81:461
Zhang S, Li X, Zong M, Zhu X, Wang R (2017) Efficient kNN classification with different numbers of nearest neighbors. IEEE Trans Neural Networks Learn Syst 29:1774–1784
Segev N, Harel M, Mannor S et al (2017) Learn on source, refine on target: a model transfer learning framework with random forests. IEEE Trans Pattern Anal Mach Intell 39:1811–1824
Gu B, Sheng VS, Tay KY et al (2017) Cross validation through two-dimensional solution surface for cost-sensitive SVM. IEEE Trans Pattern Anal Mach Intell 39:1103–1121
Kuhn M, Wing J, Weston S et al (2021) Classification and regression training. https://github.com/topepo/caret/BugReports. Accessed 1 Dec 2021
Robin, Xavier, Natacha Turck AH (2021) Display and analyze ROC curves version. http://expasy.org/tools/pROC/. Accessed 1 Dec 2021
Rogers SL, Tikellis G, Cheung N et al (2008) Retinal arteriolar caliber predicts incident retinopathy. Diabetes Care 31:761–763
Cunha-Vaz J, Ribeiro L, Costa M et al (2017) Diabetic retinopathy phenotypes of progression to macular edema: pooled analysis from independent longitudinal studies of up to 2 years’ duration. Invest Ophthalmol Vis Sci 58:206–210
Bearse MA, Adams AJ, Han Y et al (2006) A multifocal electroretinogram model predicting the development of diabetic retinopathy. Prog Retin Eye Res 25:425–448
Blighe K, Gurudas S, Lee Y et al (2020) Diabetic retinopathy environment-wide association study (EWAS) in NHANES 2005–2008. J Clin Med 9:1–18
Rohan TE, Frost CD, Wald NJ (1989) Prevention of blindness by screening for diabetic retinopathy: a quantitative assessment. Br Med J 299:1198–1201
Zhao Y, Singh RP (2018) The role of anti-vascular endothelial growth factor (anti-VEGF) in the management of proliferative diabetic retinopathy. Drugs Context 7:1–10
Xu Y, Wang A, Lin X et al (2020) Global burden and gender disparity of vision loss associated with diabetes retinopathy. Acta Ophthalmol 99:431–440
Dixon RF, Zisser H, Layne JE et al (2020) A virtual type 2 diabetes clinic using continuous glucose monitoring and endocrinology visits. J Diabetes Sci Technol 14:908–911
Downing J, Bollyky J, Schneider J (2017) Use of a connected glucose meter and certified diabetes educator coaching to decrease the likelihood of abnormal blood glucose excursions: the livongo for diabetes program. J Med Internet Res 19:2017
Funding
This study was supported by the National Natural Science Foundation of China (82220108017, 82141128); The Capital Health Research and Development of Special (2020–1-2052); Science & Technology Project of Beijing Municipal Science & Technology Commission (Z201100005520045, Z181100001818003).
Author information
Authors and Affiliations
Contributions
W.B. Wei, H.Y Li, and L. Dong designed the study. H.Y Li, L. Dong, and R.H Zhang wrote the manuscript. H.Y Li, C.Y Yu, W.D Zhou, H.T Wu, and Y.T Li collected the data and conducted the analyses. W.B. Wei edited and revised the manuscript. All authors have approved the submitted version and agreed with the contributions declarations.
Corresponding author
Ethics declarations
Ethics approval and consent to participate
Ethics approval and informed consent were not required for this study because of public accessibility to the data.
Consent for publication
Not applicable.
Competing interests
The authors declare no competing interests.
Additional information
Publisher's note
Springer Nature remains neutral with regard to jurisdictional claims in published maps and institutional affiliations.
Supplementary Information
Below is the link to the electronic supplementary material.
Rights and permissions
Springer Nature or its licensor holds exclusive rights to this article under a publishing agreement with the author(s) or other rightsholder(s); author self-archiving of the accepted manuscript version of this article is solely governed by the terms of such publishing agreement and applicable law.
About this article
Cite this article
Li, HY., Dong, L., Zhou, WD. et al. Development and validation of medical record-based logistic regression and machine learning models to diagnose diabetic retinopathy. Graefes Arch Clin Exp Ophthalmol 261, 681–689 (2023). https://doi.org/10.1007/s00417-022-05854-9
Received:
Revised:
Accepted:
Published:
Issue Date:
DOI: https://doi.org/10.1007/s00417-022-05854-9