Abstract
Purpose
Clinical evaluation of the shoulder range of motion (RoM) may vary significantly depending on the surgeon. We aim to validate an automatic shoulder RoM measurement system associating image acquisition by an RGB-D (red/green/blue–depth) video camera to an artificial intelligence (AI) algorithm.
Methods
Thirty healthy volunteers were included. A 3D RGB-D sensor that simultaneously generated a colour image and a depth map was used. Then, an open-access convolutional neural network algorithm that was programmed for shoulder recognition provided a 3D motion measure. Each volunteer adopted a randomized position successively. For each position, two observers made a visual (EyeREF) and goniometric measurement (GonioREF), blind to the automated software which was implemented by an orthopaedic surgeon. We evaluated the inter-tester intra-class correlation (ICC) between observers and the concordance correlation coefficient (CCC) between the three methods.
Results
For manual evaluations EyeREF and GonioREF, ICC remained constantly excellent for the widest motions in the vertical plane (i.e., abduction and flexion). It was very good for ER1 and IR2 and fairly good for adduction, extension, and ER2. Differences between the measurements’ means of EyeREF and shoulder RoM was significant for all motions. Compared to GonioREF, shoulder RoM provided similar results for abduction, adduction, and flexion and EyeREF provided similar results for adduction, ER1, and ER2. The three methods showed an overall good to excellent CCC. The mean bias between the three methods remained under 10° and clinically acceptable.
Conclusion
RGB-D/AI combination is reliable in measuring shoulder RoM in consultation, compared to classic goniometry and visual observation.





Similar content being viewed by others
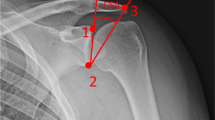
Data availability
N/A.
Code availability
The used code is open source (https://github.com/princeton-vl/pose-hg-train).
References
Conboy VB, Morris RW, Kiss J, Carr AJ (1996) An evaluation of the Constant-Murley shoulder assessment. J. Bone Joint Surg. Br. 78:229–232
Cools AM, De Wilde L, Van Tongel A et al (2014) Measuring shoulder external and internal rotation strength and range of motion: comprehensive intra-rater and inter-rater reliability study of several testing protocols. J. Shoulder Elb. Surg. 23:1454–1461. https://doi.org/10.1016/j.jse.2014.01.006
Mullaney MJ, McHugh MP, Johnson CP, Tyler TF (2010) Reliability of shoulder range of motion comparing a goniometer to a digital level. Physiother Theory Pract 26:327–333. https://doi.org/10.3109/09593980903094230
Riddle DL, Rothstein JM, Lamb RL (1987) Goniometric reliability in a clinical setting. Shoulder measurements. Phys Ther 67:668–673. https://doi.org/10.1093/ptj/67.5.668
Williams JG, Callaghan M (1990) Comparison of visual estimation and goniometry in determination of a shoulder joint angle. Physiotherapy 76:655–657. https://doi.org/10.1016/S0031-9406(10)63103-3
Corazza S, Gambaretto E, Mündermann L, Andriacchi TP (2010) Automatic generation of a subject-specific model for accurate markerless motion capture and biomechanical applications. IEEE Trans Biomed Eng 57:806–812. https://doi.org/10.1109/TBME.2008.2002103
Hayes K, Walton JR, Szomor ZR, Murrell GA (2001) Reliability of five methods for assessing shoulder range of motion. Aust J Physiother 47:289–294. https://doi.org/10.1016/s0004-9514(14)60274-9
Hanney WJ, Kolber MJ, Marshall JS (2011) The reliability of clinical measurements designed to quantify shoulder mobility. Physical Therapy Reviews 16:413–422. https://doi.org/10.1179/1743288X11Y.0000000023
Terwee CB, de Winter AF, Scholten RJ et al (2005) Interobserver reproducibility of the visual estimation of range of motion of the shoulder. Arch Phys Med Rehabil 86:1356–1361. https://doi.org/10.1016/j.apmr.2004.12.031
Matsen FA, Lauder A, Rector K et al (2016) Measurement of active shoulder motion using the Kinect, a commercially available infrared position detection system. J Shoulder Elbow Surg 25:216–223. https://doi.org/10.1016/j.jse.2015.07.011
Newell A, Yang K, Deng J (2016) Stacked hourglass networks for human pose estimation. https://arxiv.org/abs/160306937
Simovitch R, Flurin P-H, Wright T et al (2018) Quantifying success after total shoulder arthroplasty: the minimal clinically important difference. J. Shoulder Elb. Surg. 27:298–305. https://doi.org/10.1016/j.jse.2017.09.013
Clark RA, Pua Y-H, Fortin K et al (2012) Validity of the Microsoft Kinect for assessment of postural control. Gait Posture 36:372–377. https://doi.org/10.1016/j.gaitpost.2012.03.033
Partik BL, Stadler A, Schamp S et al (2002) 3D versus 2D ultrasound: accuracy of volume measurement in human cadaver kidneys. Invest Radiol 37:489–495. https://doi.org/10.1097/01.RLI.0000023573.59066.43
van de Pol RJ, van Trijffel E, Lucas C (2010) Inter-rater reliability for measurement of passive physiological range of motion of upper extremity joints is better if instruments are used: a systematic review. J Physiother 56:7–17. https://doi.org/10.1016/s1836-9553(10)70049-7
Wilson JD, Khan-Perez J, Marley D et al (2017) Can shoulder range of movement be measured accurately using the Microsoft Kinect sensor plus Medical Interactive Recovery Assistant (MIRA) software? J Shoulder Elbow Surg 26:e382–e389. https://doi.org/10.1016/j.jse.2017.06.004
Huber ME, Seitz AL, Leeser M, Sternad D (2015) Validity and reliability of Kinect skeleton for measuring shoulder joint angles: a feasibility study. Physiotherapy 101:389–393. https://doi.org/10.1016/j.physio.2015.02.002
Zulkarnain RF, Kim G-Y, Adikrishna A et al (2017) Digital data acquisition of shoulder range of motion and arm motion smoothness using Kinect v2. J Shoulder Elbow Surg 26:895–901. https://doi.org/10.1016/j.jse.2016.10.026
Çubukçu B, Yüzgeç U, Zileli R, Zileli A (2020) Reliability and validity analyzes of Kinect V2 based measurement system for shoulder motions. Med. Eng. Phys. 76:20–31. https://doi.org/10.1016/j.medengphy.2019.10.017
Author information
Authors and Affiliations
Contributions
Conceptualization, MO Gauci; methodology: MO Gauci; software: A Murienne and M Urvoy; validation: MO Gauci and M Olmos; formal analysis: M Urvoy and MO Gauci; investigation: M Olmos, C Cointat, and PE Chammas; data curation: M Olmos; writing—original draft preparation: MO Gauci and M Olmos; writing—review and editing: MO Gauci; visualization: JF Gonzalez; supervision: MO Gauci; project administration: N Bronsard and MO Gauci.
Corresponding author
Ethics declarations
Ethics approval
The study was approved by the Institutional Review Board (or Ethics Committee) of Institut Universitaire Locomoteur et du Sport (protocol IRB: IULS202104). The study was conducted according to the guidelines of the Declaration of Helsinki.
Consent to participate
Informed consent was obtained from all individual participants included in the study.
Consent for publication
All authors have read and agreed to the published version of the manuscript.
Competing interests
Marc-Olivier Gauci is a consultant of Imascap (Now part of Stryker), and Manuel Urvoy is an employee of Imascap. The other authors and their family do not declare any conflict of interest for this study.
Additional information
Publisher’s note
Springer Nature remains neutral with regard to jurisdictional claims in published maps and institutional affiliations.
Rights and permissions
Springer Nature or its licensor (e.g. a society or other partner) holds exclusive rights to this article under a publishing agreement with the author(s) or other rightsholder(s); author self-archiving of the accepted manuscript version of this article is solely governed by the terms of such publishing agreement and applicable law.
About this article
Cite this article
Gauci, MO., Olmos, M., Cointat, C. et al. Validation of the shoulder range of motion software for measurement of shoulder ranges of motion in consultation: coupling a red/green/blue-depth video camera to artificial intelligence. International Orthopaedics (SICOT) 47, 299–307 (2023). https://doi.org/10.1007/s00264-022-05675-9
Received:
Accepted:
Published:
Issue Date:
DOI: https://doi.org/10.1007/s00264-022-05675-9